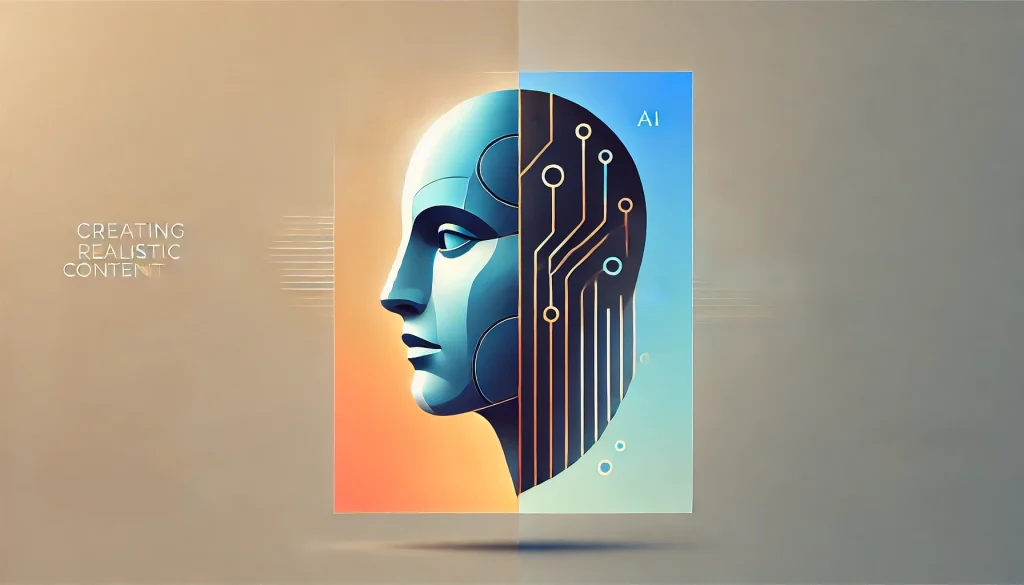
GANs: Creating Realistic Content with AI
In the ever-evolving landscape of artificial intelligence (AI), Generative Adversarial Networks (GANs) have emerged as one of the most groundbreaking innovations. With their ability to create highly realistic content, GANs are revolutionizing various industries, from art and entertainment to healthcare and security. But what exactly are GANs, and how do they manage to produce such astonishing results? This blog will dive deep into the world of GANs, exploring their mechanisms, applications, challenges, and future potential. Whether you’re a college student, a young professional, or simply someone curious about AI, this comprehensive guide will provide you with a thorough understanding of GANs and their transformative power.
What Are GANs?
A Brief Overview
Generative Adversarial Networks, commonly known as GANs, are a class of AI algorithms used in unsupervised machine learning. They were introduced by Ian Goodfellow and his colleagues in 2014. The core idea behind GANs is relatively simple yet ingenious: two neural networks, a generator and a discriminator, are pitted against each other in a zero-sum game.
How GANs Work
The generator creates fake data that resembles the real data it’s been trained on, while the discriminator evaluates this data and attempts to distinguish between real and fake inputs. Over time, both networks improve, with the generator producing increasingly realistic content and the discriminator becoming better at spotting the fakes. This adversarial process continues until the generated data is almost indistinguishable from the real data.
The Components
- Generator: This neural network creates new data instances. For example, in image generation, it might start with random noise and transform it into a photo-realistic image.
- Discriminator: This neural network evaluates the generated data against real data, providing feedback to the generator to improve its output.
The interaction between these two networks creates a feedback loop, enabling the generator to produce highly realistic content over time.
The Science Behind GANs
Mathematical Foundation
At the heart of GANs lies game theory. The generator and discriminator are locked in a minimax game, where the generator aims to minimize its loss (producing more realistic data), while the discriminator aims to maximize its ability to distinguish between real and fake data. Mathematically, this can be expressed using the following loss functions:
- Generator Loss: This measures how well the generator is tricking the discriminator.
- Discriminator Loss: This measures how well the discriminator is distinguishing between real and fake data.
The training process involves optimizing these loss functions using gradient descent, a common method in machine learning.
Training Process
Training GANs is notoriously challenging. It requires balancing the generator and discriminator’s performance, ensuring neither network outpaces the other too quickly. If the discriminator becomes too powerful, it easily identifies fakes, providing little useful feedback to the generator. Conversely, if the generator outpaces the discriminator, the latter fails to learn and the overall system collapses.
To address these challenges, researchers have developed various techniques, such as:
- Feature Matching: Modifying the generator’s loss to match statistics of real data.
- Mini-Batch Discrimination: Improving the discriminator’s robustness by using batches of data.
- Historical Averaging: Regularizing the training process by averaging historical parameters.
Applications of GANs
Creative Industries
GANs have made a significant impact in creative fields. Artists and designers use GANs to generate new artwork, explore novel design concepts, and even create music. For instance, GANs can create paintings in the style of famous artists, generate realistic human faces for character design, and produce music tracks that mimic specific genres.
Entertainment
In the entertainment industry, GANs are revolutionizing the way content is created. They are used in movie production to generate realistic visual effects, enhance video game graphics, and even create entirely new characters. GANs can also be used to restore old films by filling in missing frames and improving image quality.
Healthcare
In healthcare, GANs are being utilized for medical imaging. They can generate high-resolution images from low-resolution inputs, assisting in diagnostics and treatment planning. GANs also help in creating synthetic medical data, which can be used for research and training purposes without compromising patient privacy.
Security
GANs have applications in security, particularly in the field of cybersecurity. They can be used to generate realistic phishing emails for training purposes, helping organizations prepare for potential cyber-attacks. Additionally, GANs are used to create deepfakes, which, while posing ethical concerns, also provide insights into potential security threats and the development of countermeasures.
Fashion
The fashion industry is leveraging GANs to design new clothing lines, generate fashion trends, and even create virtual models. GANs can analyze current fashion trends and generate new designs that align with popular styles, helping designers stay ahead of the curve.
Automotive
In the automotive sector, GANs are used for autonomous vehicle training. They can generate realistic driving scenarios, helping train AI models to handle various road conditions and situations. This accelerates the development of self-driving cars by providing vast amounts of training data that would be difficult to obtain otherwise.
Challenges and Limitations
Training Instability
One of the main challenges with GANs is training instability. The adversarial nature of GANs can lead to situations where one network overpowers the other, causing the training process to fail. Researchers are continually developing new techniques to stabilize training and ensure balanced progress for both networks.
Mode Collapse
Mode collapse occurs when the generator produces a limited variety of outputs, failing to capture the full diversity of the training data. This results in repetitive and less realistic content. Techniques such as mini-batch discrimination and unrolled GANs have been proposed to address this issue, but it remains a significant challenge.
Ethical Concerns
The ability of GANs to create highly realistic content raises ethical concerns, particularly regarding the creation of deepfakes. These are videos or images that convincingly depict events or people in situations that never occurred. Deepfakes can be used for malicious purposes, such as spreading misinformation, blackmail, and political manipulation. Addressing these ethical concerns requires the development of detection technologies and legal frameworks to prevent misuse.
Computational Resources
Training GANs requires significant computational resources, including powerful GPUs and large datasets. This can be a barrier for smaller organizations and individuals, limiting the accessibility and widespread adoption of GANs. However, advancements in hardware and cloud computing are gradually lowering these barriers.
Future Prospects
Advancements in Training Techniques
Future research in GANs will likely focus on developing more robust training techniques to address current challenges. This includes improving methods to stabilize training, prevent mode collapse, and enhance the overall performance of GANs. Innovations in this area will make GANs more reliable and effective for various applications.
Integration with Other AI Technologies
GANs will increasingly be integrated with other AI technologies, such as reinforcement learning and natural language processing. This integration will enable the development of more sophisticated AI systems capable of generating realistic content in multiple domains, including text, images, and audio.
Personalized Content Generation
In the future, GANs will be used to create highly personalized content tailored to individual preferences. This could revolutionize industries such as marketing and entertainment, providing consumers with customized experiences that enhance engagement and satisfaction.
Ethical and Regulatory Frameworks
As GANs become more prevalent, there will be a growing need for ethical and regulatory frameworks to ensure their responsible use. This includes developing technologies to detect and prevent malicious uses of GANs, as well as establishing legal guidelines to protect individuals and organizations from potential harm.
Conclusion
Generative Adversarial Networks represent a significant leap forward in the field of artificial intelligence. By harnessing the power of adversarial training, GANs can create incredibly realistic content, opening up new possibilities across various industries. From art and entertainment to healthcare and security, the applications of GANs are vast and transformative. However, with great power comes great responsibility. Addressing the challenges and ethical concerns associated with GANs is crucial to ensuring their positive impact on society.
As we continue to explore the potential of GANs, it’s essential to remain mindful of the ethical implications and strive for a balanced approach that leverages their capabilities for the greater good. The future of GANs is undoubtedly exciting, promising a world where AI-generated content becomes an integral part of our daily lives.
Disclaimer: This blog is intended for informational purposes only. While every effort has been made to ensure accuracy, any errors or omissions are unintentional. Please report any inaccuracies so we can correct them promptly.