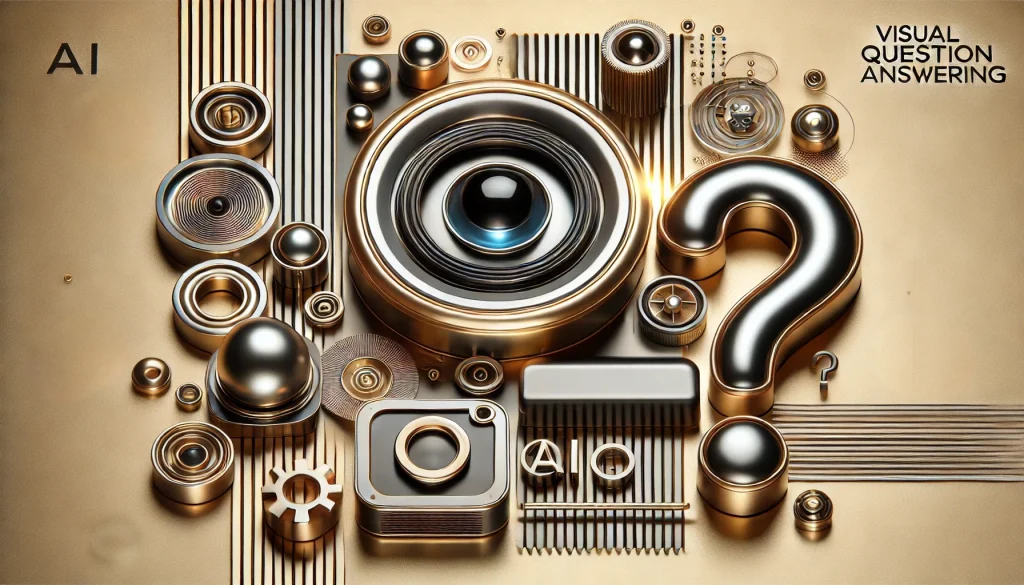
Visual Question Answering: AI That Can See and Answer
Visual Question Answering (VQA) is an exciting and rapidly growing field in artificial intelligence (AI). Imagine a system that can look at an image and answer questions about it. This capability has vast implications, from helping visually impaired individuals to enhancing security systems and creating more interactive and intelligent user experiences. In this blog, we’ll explore the fascinating world of VQA, diving into how it works, its applications, challenges, and the future of this technology. Let’s embark on this journey to understand how AI is learning to see and answer!
What is Visual Question Answering?
VQA combines computer vision and natural language processing (NLP), two critical areas in AI, to interpret visual data and provide answers to questions about that data. Essentially, it allows machines to understand images at a deeper level and respond to queries related to those images in human-like ways. This process involves several complex steps, including image recognition, semantic understanding, and context-based reasoning. VQA systems can be trained to perform a wide range of tasks, from identifying objects and their attributes in images to understanding complex scenes and generating descriptive answers.
How Does Visual Question Answering Work?
The Magic Behind VQA
The process begins with image processing. The AI system uses sophisticated algorithms to analyze the image, identify objects, and understand the scene. This stage, known as computer vision, involves techniques like convolutional neural networks (CNNs) that can detect patterns and features within the image.
Once the image is processed, the system moves on to natural language processing. Here, the AI interprets the question posed by the user. This step is crucial as it requires the system to understand not just the words but the context and intent behind the question. Advanced NLP models, such as those based on transformers, play a vital role in this phase.
Bringing It All Together
After processing the image and the question, the system combines the information to generate an answer. This involves a reasoning process where the AI correlates visual data with textual data. For instance, if the question is “What is the color of the car?” and the image shows a red car, the system needs to link the concept of “color” with the visual representation of the car and respond with “red.”
Applications of Visual Question Answering
Empowering the Visually Impaired
One of the most impactful applications of VQA is in assisting visually impaired individuals. Imagine a person who cannot see holding up their phone to an object and asking, “What is this?” The VQA system can analyze the image and provide a spoken answer, significantly enhancing the person’s ability to interact with their surroundings.
Enhancing Security Systems
In security and surveillance, VQA can play a crucial role. Systems equipped with VQA can analyze live camera feeds and answer questions about activities and objects within the scene. For example, security personnel could ask, “Is there any suspicious activity in zone 3?” and receive real-time answers based on the visual data.
Revolutionizing E-Commerce
In the e-commerce sector, VQA can revolutionize the shopping experience. Customers can upload pictures of products and ask questions like, “Is this available in blue?” or “What size is this?” This capability can make online shopping more interactive and user-friendly, bridging the gap between physical and online stores.
Educational Tools
VQA systems can also be used as educational tools. Students can upload images related to their studies and ask questions to gain a deeper understanding. For instance, a biology student could upload an image of a plant and ask, “What type of plant is this?” The VQA system can provide detailed answers, making learning more engaging and interactive.
Challenges in Visual Question Answering
Complexity of Visual Data
One of the significant challenges in VQA is the complexity of visual data. Images can contain a vast amount of information, and interpreting this data accurately is not always straightforward. Factors like lighting, angle, and occlusion can affect the system’s ability to understand the image correctly.
Understanding Context and Intent
Another challenge is understanding the context and intent behind the questions. Human language is incredibly nuanced, and interpreting questions accurately requires a deep understanding of context. For example, the question “What’s on the table?” could refer to objects on top of the table or the material of the table itself, depending on the context.
Data Requirements and Training
Training VQA systems requires vast amounts of data. The AI needs to be exposed to numerous images and questions to learn effectively. This data collection and annotation process can be time-consuming and expensive. Furthermore, the system needs to be regularly updated with new data to maintain its accuracy and relevance.
Future of Visual Question Answering
Advancements in AI and Machine Learning
The future of VQA looks promising with ongoing advancements in AI and machine learning. As algorithms become more sophisticated, we can expect VQA systems to become more accurate and efficient. Innovations in deep learning, particularly in areas like generative adversarial networks (GANs) and reinforcement learning, will likely play a significant role in enhancing VQA capabilities.
Integration with Augmented Reality
One exciting possibility is the integration of VQA with augmented reality (AR). This combination can create immersive experiences where users can interact with their environment in real-time. For instance, AR glasses equipped with VQA can provide real-time answers to questions about objects and scenes in the user’s field of view, making everyday tasks more interactive and informative.
Personalized VQA Systems
In the future, we might see the development of personalized VQA systems tailored to individual users. These systems can learn from user interactions and preferences to provide more relevant and accurate answers. For example, a personalized VQA system could recognize a user’s pet and provide detailed answers to questions about the pet’s breed, health, and care.
Ethical Considerations in Visual Question Answering
Privacy Concerns
As with any technology that involves data collection and analysis, VQA raises privacy concerns. The ability to analyze and interpret images can lead to potential misuse if not properly regulated. It’s crucial to ensure that VQA systems are designed and implemented with privacy in mind, safeguarding users’ personal data and preventing unauthorized access.
Bias and Fairness
Another ethical consideration is bias and fairness in VQA systems. AI systems can inadvertently learn and propagate biases present in the training data. For instance, if a VQA system is trained on a dataset that lacks diversity, it may not perform well for users from different backgrounds. Ensuring that VQA systems are trained on diverse and representative datasets is essential to avoid biases and ensure fairness.
Transparency and Accountability
Transparency and accountability are also critical in the development and deployment of VQA systems. Users should be aware of how their data is being used and have the ability to question and understand the decisions made by the AI. Developing mechanisms for transparency and accountability can build trust and ensure ethical use of VQA technology.
Conclusion
Visual Question Answering represents a significant leap forward in AI technology. By combining the power of computer vision and natural language processing, VQA systems can understand and interpret images in ways that were previously unimaginable. The applications of VQA are vast, ranging from assisting the visually impaired to enhancing security systems and revolutionizing e-commerce. However, the development of VQA also comes with challenges and ethical considerations that need to be addressed. As we look to the future, advancements in AI and machine learning promise to further enhance the capabilities of VQA, making it an integral part of our daily lives. Embracing this technology while ensuring ethical practices will be key to unlocking its full potential and creating a more interactive and intelligent world.
Disclaimer: The information provided in this blog is for educational purposes only and may not be exhaustive. While we strive for accuracy, there may be errors or omissions. Please report any inaccuracies so we can correct them promptly.