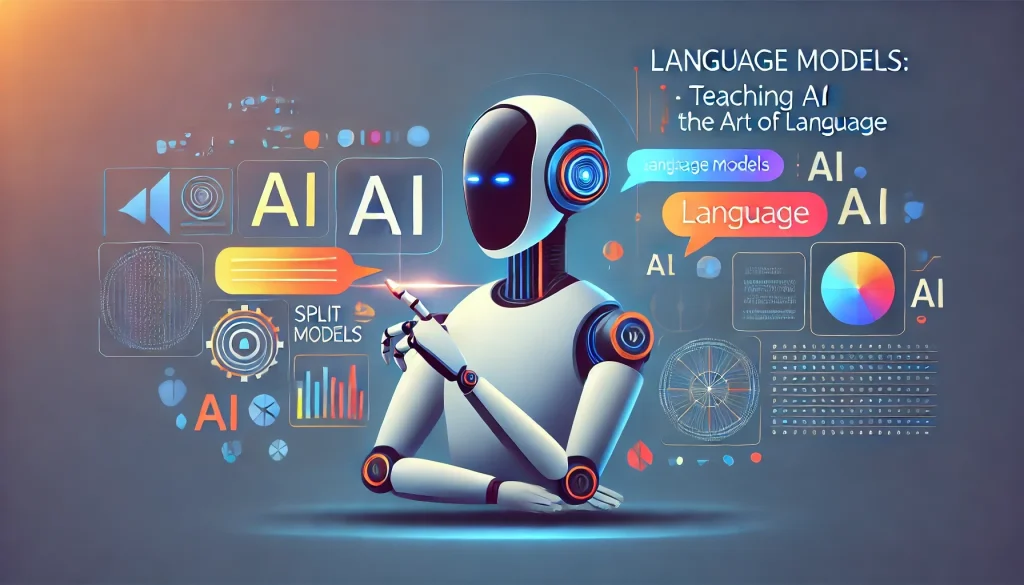
Language Models: Teaching AI the Art of Language
Language is one of humanity’s most intricate and cherished tools, enabling us to share our thoughts, feelings, and ideas. The advent of artificial intelligence (AI) has revolutionized many fields, and language is no exception. Language models, the backbone of modern AI’s linguistic capabilities, are at the forefront of this transformation. This blog delves into the fascinating world of language models, exploring their development, functionality, and impact on our daily lives.
What are Language Models?
Language models are AI systems designed to understand, generate, and manipulate human language. These models are built using large datasets containing billions of words and phrases from diverse sources, including books, articles, websites, and social media. By analyzing these datasets, language models learn the nuances of human language, enabling them to perform tasks such as text completion, translation, summarization, and even creative writing.
Historical Background
The journey of language models began decades ago with rule-based systems that relied on predefined grammar rules and vocabulary lists. However, these early models were limited in their ability to handle the complexity and variability of human language. The introduction of machine learning techniques in the late 20th century marked a significant milestone, allowing models to learn from data rather than relying solely on hard-coded rules.
The Rise of Neural Networks
Neural networks, particularly deep learning models, have revolutionized the field of natural language processing (NLP). These models consist of multiple layers of interconnected nodes, or “neurons,” that mimic the structure of the human brain. By processing vast amounts of text data, neural networks can identify patterns and relationships between words, phrases, and sentences, leading to more accurate and sophisticated language models.
Transformers: A Game-Changer in NLP
The development of the transformer architecture in 2017 marked a breakthrough in language modeling. Unlike traditional neural networks, transformers use self-attention mechanisms to weigh the importance of different words in a sentence, allowing the model to capture long-range dependencies and contextual information. This innovation paved the way for advanced language models like BERT (Bidirectional Encoder Representations from Transformers) and GPT (Generative Pre-trained Transformer).
How Do Language Models Work?
To understand how language models work, it’s essential to grasp the basic principles of machine learning and deep learning. At the core, these models use algorithms to analyze and learn from data, making predictions or generating outputs based on the patterns they identify.
Training Process
Training a language model involves feeding it large amounts of text data and adjusting its parameters to minimize errors. This process, known as “training,” typically involves multiple iterations, or epochs, during which the model refines its understanding of language. The goal is to optimize the model’s performance on specific tasks, such as predicting the next word in a sentence or generating coherent text.
Tokenization and Embeddings
Language models begin by breaking down text into smaller units called tokens, which can be words, subwords, or characters. Each token is then converted into a numerical representation, known as an embedding, which captures its meaning and context. These embeddings are crucial for the model to understand the relationships between different words and phrases.
Attention Mechanisms
Attention mechanisms are a key feature of modern language models, allowing them to focus on the most relevant parts of the input text. By assigning different weights to different tokens, attention mechanisms enable the model to prioritize important information and generate more accurate outputs. This capability is particularly useful for tasks like translation and summarization, where context and nuance are critical.
Fine-Tuning and Adaptation
Once a language model is trained on a large dataset, it can be fine-tuned for specific tasks or domains. Fine-tuning involves further training the model on a smaller, task-specific dataset, allowing it to adapt to the nuances of a particular language or subject matter. This process enhances the model’s performance and makes it more versatile and applicable to a wide range of applications.
Applications of Language Models
Language models have a wide range of applications, from everyday tasks like writing emails and creating social media content to more complex functions like legal document analysis and medical research. Here are some of the most notable applications:
Text Generation and Completion
One of the most popular uses of language models is text generation, where the model creates coherent and contextually relevant text based on a given prompt. This capability is used in various applications, including content creation, creative writing, and chatbots. Language models can also complete sentences or paragraphs, making them valuable tools for drafting documents and generating ideas.
Translation and Multilingual Support
Language models have significantly improved machine translation, enabling more accurate and natural translations between different languages. By understanding the context and nuances of both the source and target languages, these models can produce translations that are more fluent and meaningful. This technology is essential for breaking down language barriers and fostering global communication.
Summarization and Information Retrieval
Summarization involves condensing long texts into shorter, more manageable summaries while retaining the essential information. Language models excel at this task, making them valuable for news aggregation, legal document analysis, and academic research. Additionally, these models can be used for information retrieval, helping users find relevant information quickly and efficiently.
Sentiment Analysis and Opinion Mining
Sentiment analysis involves determining the emotional tone or sentiment behind a piece of text. Language models can accurately identify positive, negative, or neutral sentiments, making them useful for monitoring social media, customer reviews, and market trends. Opinion mining, a related task, involves extracting opinions and insights from large volumes of text, providing valuable information for businesses and policymakers.
Personal Assistants and Chatbots
Personal assistants like Siri, Alexa, and Google Assistant rely on language models to understand and respond to user queries. These models enable the assistants to carry out tasks such as setting reminders, answering questions, and controlling smart home devices. Chatbots, used in customer service and support, also leverage language models to provide accurate and helpful responses to user inquiries.
Challenges and Ethical Considerations
While language models have made remarkable advancements, they also present several challenges and ethical considerations that need to be addressed.
Bias and Fairness
Language models are trained on large datasets that may contain biased or unrepresentative information. As a result, these models can inadvertently perpetuate and amplify existing biases, leading to unfair or discriminatory outcomes. Ensuring fairness and reducing bias in language models is a critical area of ongoing research.
Privacy and Security
The use of language models raises concerns about privacy and security, particularly when dealing with sensitive or personal information. Ensuring that these models handle data responsibly and securely is essential to protect users’ privacy and prevent misuse.
Misinformation and Manipulation
Language models have the potential to generate realistic but false or misleading information, contributing to the spread of misinformation. Addressing this issue requires developing mechanisms to detect and mitigate false information and ensuring that language models are used responsibly.
Transparency and Accountability
The complexity of language models can make it difficult to understand how they arrive at certain decisions or outputs. Increasing transparency and accountability in the development and deployment of these models is crucial for building trust and ensuring their responsible use.
The Future of Language Models
The future of language models holds exciting possibilities, with ongoing research and development aimed at making these models more powerful, versatile, and ethical.
Continued Advancements in NLP
Researchers are continuously exploring new techniques and architectures to improve the performance and capabilities of language models. Innovations such as few-shot learning, which enables models to learn from limited data, and the integration of multimodal data, combining text, images, and audio, are expected to drive further advancements in NLP.
Enhanced Human-AI Collaboration
As language models become more sophisticated, they will play an increasingly important role in augmenting human capabilities and facilitating collaboration between humans and AI. These models can assist with complex tasks, provide valuable insights, and enable more efficient and effective communication.
Ethical AI and Responsible Use
Ensuring the ethical and responsible use of language models is a key priority for the future. This involves developing guidelines and best practices for the deployment and use of these models, as well as fostering collaboration between researchers, policymakers, and industry stakeholders to address ethical and societal implications.
Personalized and Context-Aware AI
Future language models are expected to become more personalized and context-aware, tailoring their responses and interactions to individual users’ needs and preferences. This will enhance the user experience and make AI more relevant and useful in various contexts.
Conclusion
Language models have transformed the way we interact with technology, enabling AI to understand, generate, and manipulate human language in unprecedented ways. From text generation and translation to sentiment analysis and personal assistants, these models have a wide range of applications that are reshaping industries and enhancing our daily lives. However, the development and deployment of language models also present significant challenges and ethical considerations that must be addressed to ensure their responsible and fair use. As we look to the future, continued advancements in NLP, enhanced human-AI collaboration, and a focus on ethical AI will drive the evolution of language models, unlocking new possibilities and opportunities for innovation.
Disclaimer: This blog is for informational purposes only and reflects the current state of knowledge as of the publication date. While every effort has been made to ensure the accuracy of the information provided, we encourage readers to report any inaccuracies or issues so we can correct them promptly.