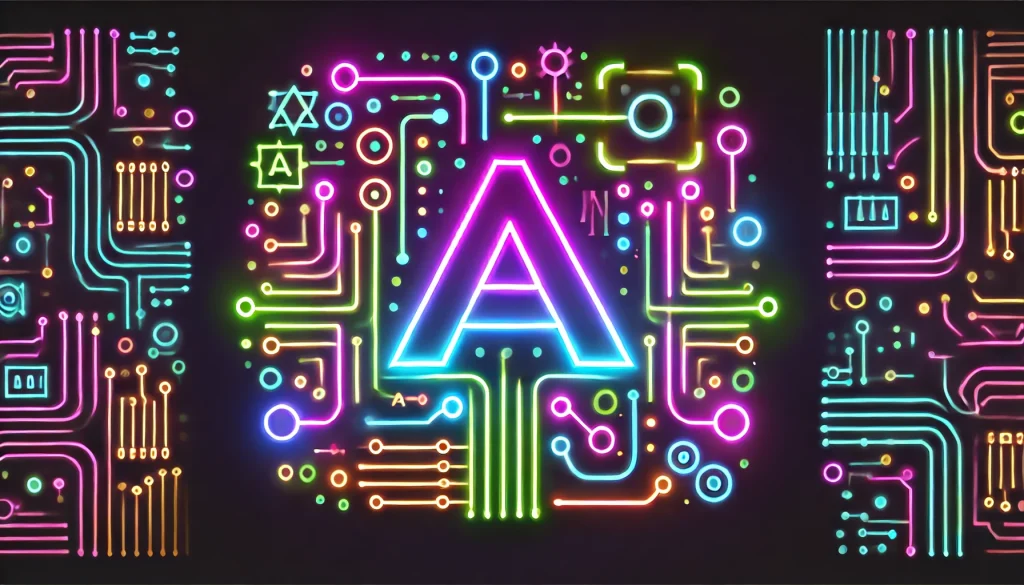
Understanding Bias in AI: How Algorithms Can Be Unfair
In the age of artificial intelligence, we’re surrounded by algorithms that shape our daily lives in ways we might not even realize. From the content we see on social media to the job applications that make it past initial screenings, AI is making decisions that impact us all. But have you ever stopped to wonder if these algorithms are always fair? Or if they might be perpetuating biases that exist in our society? Today, we’re diving deep into the world of AI bias – a topic that’s as fascinating as it is crucial to understand in our increasingly digital world.
The Hidden Influence of AI in Our Lives
Let’s start by taking a moment to consider just how pervasive AI has become in our everyday experiences. Every time you open your favorite social media app, an algorithm is hard at work deciding which posts to show you first. When you apply for a loan, AI might be involved in determining your creditworthiness. Even in healthcare, AI systems are being used to assist in diagnosing diseases and recommending treatment plans.
These AI-driven systems are designed to make our lives easier and more efficient. They can process vast amounts of data in seconds, spotting patterns and making predictions that would take humans far longer to achieve. In many ways, this is incredibly beneficial. AI can help doctors detect cancer earlier, enable banks to prevent fraud more effectively, and allow companies to personalize their services to better meet our needs.
But here’s the catch: these algorithms, no matter how sophisticated, are ultimately created by humans. And humans, as we all know, come with their own set of biases and preconceptions about the world. When these biases find their way into AI systems – whether intentionally or not – the consequences can be far-reaching and sometimes deeply unfair.
What Exactly Is AI Bias?
Defining the Problem
Before we delve deeper, let’s clarify what we mean by AI bias. In essence, AI bias occurs when an algorithm produces results that are systematically prejudiced due to erroneous assumptions in the machine learning process. This can lead to unfair outcomes that disproportionately impact certain groups of people based on characteristics like race, gender, age, or socioeconomic status.
It’s important to understand that AI doesn’t develop these biases on its own. The bias typically stems from the data used to train the AI, the way the algorithm is designed, or the context in which it’s deployed. In other words, AI bias is often a reflection of existing societal biases that have been unintentionally baked into the system.
Types of AI Bias
AI bias can manifest in various forms. Some common types include:
- Sample bias: This occurs when the data used to train the AI isn’t representative of the population it will be used on. For example, if a facial recognition system is trained primarily on images of light-skinned faces, it may perform poorly when trying to recognize people with darker skin tones.
- Prejudice bias: This happens when societal prejudices are reflected in the training data or the way the algorithm is designed. For instance, an AI system for screening job applicants might be biased against women if it’s trained on historical hiring data from a male-dominated industry.
- Measurement bias: This type of bias arises when the data used to train the AI doesn’t accurately measure what it’s supposed to. An example would be using arrest rates as a proxy for crime rates, which could lead to overpolicing in certain communities.
- Algorithm bias: Sometimes, the bias is inherent in the algorithm itself, regardless of the data it’s trained on. This could be due to the way the problem is framed or the specific techniques used to solve it.
Understanding these different types of bias is crucial because it helps us identify where things can go wrong in the AI development process and how we might go about fixing them.
Real-World Examples of AI Bias
To truly grasp the impact of AI bias, let’s look at some real-world examples where algorithmic unfairness has had significant consequences.
Facial Recognition Failures
One of the most widely publicized instances of AI bias involves facial recognition technology. In 2018, researchers Joy Buolamwini and Timnit Gebru found that several commercial facial recognition systems had much higher error rates for darker-skinned women compared to lighter-skinned men. In some cases, the error rate for darker-skinned women was nearly 35% higher. This discrepancy was traced back to training datasets that were overwhelmingly composed of images of light-skinned males.
The implications of this bias are serious. Facial recognition is increasingly being used in law enforcement, airport security, and even for unlocking smartphones. Inaccurate results could lead to wrongful arrests, travel disruptions, or simply daily frustrations for certain groups of people.
Biased Hiring Algorithms
In 2018, Amazon made headlines when it was revealed that their AI-powered hiring tool was biased against women. The system, which was designed to review job applications and rate candidates, had been trained on resumes submitted to the company over a 10-year period. Because the tech industry has historically been male-dominated, the AI learned to prefer male candidates and even penalized resumes that included the word “women’s,” as in “women’s chess club captain.”
This case highlights how historical biases can be perpetuated and even amplified by AI systems if we’re not careful. Amazon eventually scrapped the tool, but it serves as a cautionary tale about the potential for AI to reinforce existing inequalities in the workplace.
Discriminatory Lending Practices
AI is increasingly being used in the financial sector to determine creditworthiness and make lending decisions. However, these systems can sometimes perpetuate historical patterns of discrimination. In 2019, researchers found that mortgage approval algorithms were more likely to reject minority applicants than white applicants with similar financial profiles.
The problem here is that if the AI is trained on historical lending data, it may pick up on past discriminatory practices and continue to apply them. This can create a vicious cycle where certain groups are systematically denied access to financial resources, making it harder for them to improve their economic situation.
These examples illustrate how AI bias can have real, tangible impacts on people’s lives. They underscore the importance of carefully examining our AI systems for potential biases and taking steps to mitigate them.
The Root Causes of AI Bias
Now that we’ve seen how AI bias can manifest in the real world, let’s dig into the underlying causes. Understanding where bias comes from is the first step in figuring out how to prevent it.
Biased Training Data
One of the primary sources of AI bias is the data used to train the algorithms. Machine learning models learn by analyzing vast amounts of data and identifying patterns within it. If this training data contains biases or is not representative of the entire population, the AI will likely inherit these biases.
For example, if a medical AI is trained primarily on data from male patients, it may not be as effective at diagnosing conditions in female patients. Similarly, if a language model is trained on text from the internet, it may pick up on and perpetuate racist or sexist language patterns that are prevalent online.
Historical Bias
Historical bias occurs when the AI system reflects past societal biases that are present in the training data. This is particularly problematic when using AI in fields where there has been historical discrimination, such as hiring, lending, or criminal justice.
For instance, if an AI system is trained on historical arrest data to predict future crime, it may disproportionately flag minority neighborhoods as high-risk areas. This is because these areas may have been over-policed in the past, leading to higher arrest rates that don’t necessarily reflect higher crime rates.
Lack of Diversity in AI Development
Another significant factor contributing to AI bias is the lack of diversity in the teams developing these systems. The AI field is predominantly male and has a notable lack of racial diversity. This homogeneity can lead to blind spots in the development process, where potential biases are overlooked simply because the developers don’t have the lived experiences to recognize them.
For example, a team of predominantly male developers might not think to test their facial recognition system on a diverse range of skin tones and gender presentations. Or they might not consider how their algorithm could disadvantage certain groups when applied in the real world.
Complexity and Opacity of AI Systems
As AI systems become more complex, it becomes increasingly difficult to understand how they arrive at their decisions. This “black box” nature of many AI algorithms can make it challenging to identify and correct biases. When we can’t see the reasoning behind an AI’s decision, it’s harder to spot when that decision is unfair or based on biased assumptions.
This opacity is particularly concerning when AI is used to make important decisions about people’s lives, such as whether they get a job, a loan, or are granted parole. If we can’t explain how these decisions are being made, it becomes difficult to ensure they’re fair and to hold the system accountable when they’re not.
The Consequences of Unchecked AI Bias
Perpetuating and Amplifying Societal Inequalities
One of the most significant dangers of AI bias is its potential to reinforce and even exacerbate existing societal inequalities. When biased AI systems are deployed at scale, they can have far-reaching effects that disproportionately impact already marginalized groups.
For instance, if biased hiring algorithms are widely adopted, they could systematically exclude certain groups from job opportunities across entire industries. This not only affects individuals’ livelihoods but can also lead to a lack of diversity in the workforce, which in turn can perpetuate biases in product development and decision-making.
Similarly, biased AI in financial services could make it harder for certain groups to access loans or financial products, limiting their economic opportunities and widening the wealth gap. In healthcare, biased AI could lead to disparities in diagnosis and treatment, potentially exacerbating health inequalities.
Erosion of Trust in AI and Technology
As more cases of AI bias come to light, there’s a risk of eroding public trust in AI and technology more broadly. This could lead to resistance against adopting potentially beneficial AI technologies, slowing down progress in fields where AI could make significant positive contributions.
Moreover, if people lose faith in the fairness of AI systems, it could undermine the legitimacy of institutions that rely on these systems. For example, if the public believes that AI-assisted judicial decisions are biased, it could decrease trust in the justice system as a whole.
Legal and Ethical Implications
Biased AI systems also raise significant legal and ethical questions. In many jurisdictions, it’s illegal to discriminate based on protected characteristics like race, gender, or age. If an AI system is found to be making biased decisions, the organizations using it could face legal consequences.
There are also broader ethical concerns about the use of potentially biased AI in high-stakes decision-making. Is it fair to let an algorithm decide who gets a job or a loan? How do we ensure accountability when AI systems make mistakes or unfair decisions? These are complex questions that society will need to grapple with as AI becomes more prevalent.
Strategies for Mitigating AI Bias
While the challenges posed by AI bias are significant, they’re not insurmountable. Here are some strategies that researchers, developers, and organizations are using to address this issue:
Diverse and Representative Training Data
One of the most straightforward ways to reduce bias is to ensure that the data used to train AI systems is diverse and representative. This means actively seeking out data from underrepresented groups and ensuring that the training set reflects the diversity of the population the AI will be used on.
For example, in facial recognition, this could involve creating datasets that include a wide range of skin tones, ages, and gender presentations. In natural language processing, it might mean training on texts from diverse authors and cultures.
Algorithmic Fairness Techniques
Computer scientists are developing various techniques to promote fairness in AI algorithms. These include:
- Pre-processing techniques: These involve modifying the training data to remove biases before the model is trained.
- In-processing techniques: These involve modifying the learning algorithm itself to ensure it produces fair outcomes.
- Post-processing techniques: These involve adjusting the model’s outputs to achieve fairness after it has been trained.
Each of these approaches has its strengths and weaknesses, and the best choice often depends on the specific context and requirements of the AI system.
Diverse AI Development Teams
Increasing diversity in AI development teams can help bring different perspectives and experiences to the table, making it more likely that potential biases will be identified and addressed early in the development process.
This doesn’t just mean diversity in terms of gender and race, but also diversity of backgrounds and disciplines. Including ethicists, social scientists, and domain experts alongside computer scientists and engineers can lead to more holistic and fair AI systems.
Transparency and Explainability
Making AI systems more transparent and explainable can help in identifying and addressing biases. This involves developing techniques that allow us to understand how AI systems arrive at their decisions, often referred to as “explainable AI” or “interpretable AI.”
By making the decision-making process of AI more understandable, we can more easily spot when it’s making unfair or biased judgments. This transparency also allows for greater accountability and can help build trust in AI systems.
Regular Auditing and Monitoring
Even after an AI system is deployed, it’s crucial to continually monitor its performance for signs of bias. This involves regularly auditing the system’s outputs across different demographic groups and contexts to ensure it’s not producing unfair results.
Some organizations are also exploring the use of “bias bounties,” similar to bug bounties in cybersecurity, where individuals are rewarded for identifying biases in AI systems.
The Role of Regulation and Policy
As AI becomes more prevalent in our lives, there’s growing recognition that regulation and policy have important roles to play in addressing AI bias.
Emerging AI Regulations
Several jurisdictions are beginning to introduce regulations specifically aimed at ensuring fairness and preventing discrimination in AI systems. For example, the European Union’s proposed AI Act includes requirements for high-risk AI systems to be tested for bias before they’re deployed.
In the United States, various states and cities have introduced legislation around the use of AI in areas like hiring and criminal justice. These regulations often require organizations to assess their AI systems for bias and provide explanations for AI-assisted decisions.
Ethical Guidelines and Standards
Various organizations and industry groups are developing ethical guidelines and standards for AI development. These often include principles around fairness, transparency, and accountability. While these guidelines are typically voluntary, they can help set industry norms and best practices.
The Need for Interdisciplinary Collaboration
Addressing AI bias effectively requires collaboration across disciplines. Policymakers need to work with technologists to craft regulations that are both effective and technically feasible. Ethicists and social scientists need to be involved to ensure that our approach to AI fairness aligns with broader societal values and goals.
The Future of Fair AI
As we look to the future, it’s clear that addressing bias in AI will remain a crucial challenge. But it’s also an opportunity to create AI systems that are not just powerful, but also fair and beneficial to all members of society.
Ongoing Research and Innovation
Research into AI fairness is a rapidly evolving field. Scientists are continually developing new techniques for detecting and mitigating bias, as well as creating AI systems that are inherently more fair and robust.
One promising area of research is in “de-biasing” techniques that can help remove unfair biases from existing AI systems. Another is the development of AI systems that can actively detect and correct their own biases over time.
Changing Mindsets in AI Development
There’s a growing recognition in the AI community that fairness and ethics need to be central considerations in AI development, not afterthoughts. This shift in mindset is leading to changes in how AI systems are designed, developed, and deployed.
Many organizations are now incorporating “ethics by design” principles, where potential ethical issues and biases are considered from the very beginning of the development process. This proactive approach can help prevent biases from being baked into AI systems in the first place.
The Importance of Public Awareness and Education
As AI becomes more prevalent in our lives, it’s crucial that the general public becomes more AI-literate. Understanding the basics of how AI works, its potential benefits and risks, and how to critically evaluate AI-assisted decisions will be important skills for everyone in the AI age.
This education needs to start early and continue throughout our lives. Schools, universities, and public institutions all have roles to play in building this AI literacy.
Conclusion
As we’ve explored in this blog post, bias in AI is a complex and multifaceted issue. It stems from various sources, including biased training data, lack of diversity in AI development, and the inherent complexity of AI systems. The consequences of unchecked AI bias can be severe, perpetuating and even amplifying societal inequalities.
However, there’s reason for optimism. Researchers, developers, policymakers, and ethicists are working hard to address these challenges. From developing more diverse training datasets to creating new algorithmic fairness techniques, from increasing diversity in AI teams to implementing robust regulations, efforts are underway on multiple fronts to make AI fairer.
Building fair AI systems isn’t just a technical challenge – it’s a societal one. It requires us to grapple with difficult questions about fairness, accountability, and the role of technology in our lives. But by facing these challenges head-on, we have the opportunity to create AI systems that not only avoid perpetuating biases but actively help to create a more equitable world.
As we move forward into an AI-powered future, let’s commit to making it a fair one. By staying informed, asking critical questions, and demanding accountability from the organizations developing and deploying AI systems, we can all play a part in shaping a future where AI works for everyone.
The journey towards fair AI is ongoing, and there will undoubtedly be challenges along the way. But with continued research, innovation, collaboration, and a commitment to ethical principles, we can harness the power of AI to create a more just and equitable world for all.
Disclaimer: This blog post is intended to provide a general overview of AI bias and its implications. While we strive for accuracy, the field of AI is rapidly evolving, and new developments may have occurred since the time of writing. We encourage readers to consult the latest research and expert opinions for the most up-to-date information on this topic. If you notice any inaccuracies in this post, please report them so we can correct them promptly.