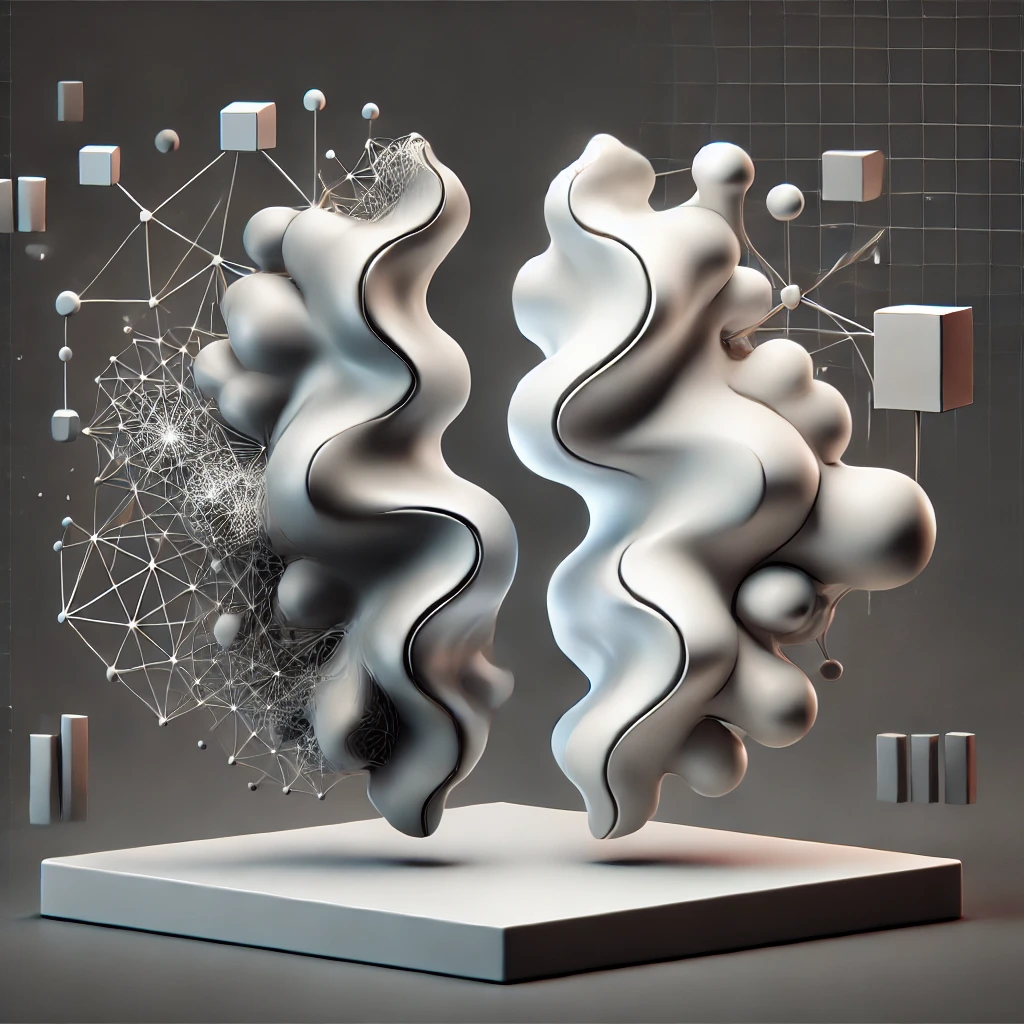
The Basics of Generative Adversarial Networks (GANs): Unleashing AI’s Creative Potential
Have you ever wondered how artificial intelligence can create stunningly realistic images, videos, or even music that never existed before? Welcome to the fascinating world of Generative Adversarial Networks, or GANs for short. In this blog post, we’re going to dive deep into the basics of GANs, exploring how these remarkable AI systems work their magic and why they’re revolutionizing the field of artificial intelligence.
What Are Generative Adversarial Networks?
The AI Artists of Our Time
Imagine two artists locked in a constant battle of creativity and critique. One artist, let’s call them the “Creator,” is always striving to produce masterpieces that look as real as possible. The other artist, we’ll dub the “Critic,” has an eagle eye for spotting fakes and tirelessly scrutinizes the Creator’s work. This artistic duel, believe it or not, is essentially what happens inside a Generative Adversarial Network.
GANs are a type of machine learning model introduced by Ian Goodfellow and his colleagues in 2014. They consist of two neural networks – a generator (our Creator) and a discriminator (our Critic) – that are trained simultaneously through adversarial training. The generator creates new data instances, while the discriminator evaluates them for authenticity. These two networks are locked in a continuous game, each becoming better at its job as training progresses.
The Dance of Creation and Criticism
The beauty of GANs lies in their adversarial nature. As the generator improves at creating realistic data, the discriminator must become more sophisticated in detecting fakes. Conversely, as the discriminator gets better at spotting generated data, the generator must up its game to produce even more convincing outputs. This ongoing competition drives both networks to improve continuously, resulting in increasingly realistic and high-quality generated data.
How Do GANs Work?
The Generator: The Master of Illusion
At the heart of every GAN is the generator network. Its job is to take random noise as input and transform it into something that resembles the training data. For example, if we’re training a GAN on a dataset of cat images, the generator’s task is to create new, realistic-looking cat images from scratch.
The generator starts with no knowledge of what a cat looks like. It begins by producing random noise, which the discriminator easily identifies as fake. But with each iteration, the generator learns from its mistakes and gradually improves its output. It’s like an artist starting with random brush strokes and slowly refining their technique until they can paint photorealistic cats.
The Discriminator: The Relentless Detective
Working in tandem with the generator is the discriminator network. Its role is to distinguish between real data from the training set and fake data produced by the generator. The discriminator is essentially a binary classifier, outputting a probability that a given input is real or fake.
As training progresses, the discriminator becomes increasingly adept at spotting generated data. It learns to pick up on subtle cues and imperfections that give away the generator’s creations. This constant improvement forces the generator to produce even more realistic outputs to have any hope of fooling the discriminator.
The Training Process: A Game of Cat and Mouse
Training a GAN is like watching an elaborate game of cat and mouse unfold. The process typically follows these steps:
- The generator creates a batch of fake data.
- The discriminator is trained on a mix of real data from the training set and fake data from the generator.
- The generator is updated based on how well it fooled the discriminator.
- Steps 1-3 are repeated many, many times.
As training continues, both networks improve their skills. The generator learns to produce data that is increasingly difficult to distinguish from real data, while the discriminator becomes better at spotting even the most subtle fakes. This adversarial training process continues until the generated data is indistinguishable from real data, or until further training no longer yields significant improvements.
Why Are GANs So Powerful?
Unleashing Creativity in AI
One of the most exciting aspects of GANs is their ability to generate new, original content. Unlike traditional machine learning models that learn to recognize patterns in existing data, GANs can create entirely new data that has never existed before. This opens up a world of possibilities in fields like art, music, and design, where AI can now act as a creative partner rather than just a tool for analysis.
Imagine an AI that can generate unique clothing designs, compose original music in the style of classical composers, or even create photorealistic images of places that don’t exist. These are all applications where GANs are making significant strides, pushing the boundaries of what we thought was possible with artificial intelligence.
Learning from Limited Data
Another powerful feature of GANs is their ability to learn from relatively small datasets. Traditional deep learning models often require massive amounts of labeled data to perform well. GANs, on the other hand, can generate new, diverse examples from a limited set of training data. This makes them particularly useful in fields where large datasets are hard to come by, such as medical imaging or rare event prediction.
That concludes the first part of the blog post. Would you like me to continue with the next sections, or would you prefer to review this part first?
Enhancing and Transforming Existing Data
GANs aren’t just good at creating new data from scratch; they’re also excellent at enhancing or transforming existing data. This capability has led to breakthroughs in image super-resolution (turning low-resolution images into high-resolution ones), style transfer (applying the style of one image to another), and even in filling in missing parts of images or videos. These applications are pushing the boundaries of what’s possible in fields like photography, film restoration, and digital art.
Applications of GANs: From Art to Science
The Art World Reimagined
One of the most visible and controversial applications of GANs has been in the art world. GANs have been used to create entirely new artworks, some of which have sold for hundreds of thousands of dollars at auction. The AI-generated portrait “Edmond de Belamy” made headlines when it sold for $432,500 at Christie’s in 2018, marking a milestone in the intersection of AI and art.
But GANs aren’t just creating static images. They’re also being used to generate music, write poetry, and even create virtual influencers on social media. These applications are raising fascinating questions about the nature of creativity and authorship in the age of AI.
Revolutionizing Entertainment and Gaming
In the world of entertainment and gaming, GANs are opening up new possibilities for content creation. They’re being used to generate realistic textures and environments in video games, create lifelike digital characters for films and TV shows, and even develop entire virtual worlds for augmented and virtual reality experiences.
Imagine a video game where every character you meet is unique, with a face you’ve never seen before, or a virtual reality experience where you can explore endlessly generating landscapes. These are the kinds of experiences that GANs are making possible.
Advancing Scientific Research
Beyond the realm of art and entertainment, GANs are proving to be powerful tools in scientific research. In drug discovery, for instance, GANs are being used to generate new molecular structures that could potentially become life-saving medications. In astronomy, they’re helping to simulate and study phenomena that are difficult or impossible to observe directly.
One particularly exciting application is in the field of climate science. Researchers are using GANs to generate high-resolution climate change projections, helping us better understand and prepare for the impacts of global warming.
Challenges and Ethical Considerations
The Dark Side of Deepfakes
While the capabilities of GANs are undoubtedly impressive, they also raise significant ethical concerns. Perhaps the most prominent of these is the issue of deepfakes – highly realistic but completely fabricated videos or audio recordings. GANs have made it possible to create convincing deepfakes that can be used to spread misinformation, manipulate public opinion, or even blackmail individuals.
As GANs continue to improve, distinguishing between real and fake content is becoming increasingly difficult. This poses serious challenges for privacy, security, and the integrity of our information ecosystem. It’s crucial that as we develop these technologies, we also work on ways to detect and mitigate their potential misuse.
Bias and Fairness in GAN-Generated Content
Another important consideration is the potential for GANs to perpetuate or even amplify biases present in their training data. If a GAN is trained on a dataset that underrepresents certain groups or contains societal biases, it may produce output that reflects and reinforces these biases.
For example, a GAN trained to generate images of business executives might predominantly produce images of white men if that’s what was most common in its training data. This could further entrench stereotypes and biases in fields where GANs are used for content creation or decision-making support.
The Future of GANs: What Lies Ahead?
Pushing the Boundaries of Realism
As GANs continue to evolve, we can expect to see even more impressive and realistic outputs. Researchers are constantly working on improving the architecture and training methods of GANs, pushing the boundaries of what’s possible. In the near future, we might see GANs capable of generating entire photorealistic videos or creating virtual environments that are indistinguishable from reality.
Combining GANs with Other AI Technologies
One exciting direction for the future of GANs is their integration with other AI technologies. For instance, combining GANs with natural language processing could lead to systems that can generate images or videos directly from textual descriptions. Or, pairing GANs with reinforcement learning might result in AI agents that can generate and interact with complex, realistic environments in real-time.
GANs in the Real World
As GANs become more sophisticated and easier to use, we’re likely to see them integrated into a wide range of everyday applications. From personalized content creation in social media apps to on-demand design tools for non-experts, GANs have the potential to make AI-powered creativity accessible to everyone.
In fields like healthcare, GANs could play a crucial role in generating synthetic data for research, protecting patient privacy while still allowing for valuable insights to be gleaned from medical datasets.
Conclusion
Generative Adversarial Networks represent a paradigm shift in artificial intelligence, moving us from systems that merely analyze and categorize existing data to those that can create entirely new content. From art and entertainment to scientific research and beyond, GANs are opening up new possibilities and challenging our understanding of creativity and intelligence.
As we continue to explore and develop this technology, it’s crucial that we remain mindful of both its incredible potential and its possible pitfalls. The ethical considerations surrounding GANs are as important as their technical capabilities, and addressing these challenges will be key to harnessing the full power of this revolutionary technology.
Whether you’re an AI enthusiast, a creative professional, or simply curious about the future of technology, keeping an eye on the world of GANs is sure to be a fascinating journey. The GAN revolution is just beginning, and its full impact on our world is yet to be seen. So buckle up and get ready – the future of AI-powered creativity is here, and it’s more exciting than we ever imagined.
Disclaimer: This blog post provides an overview of Generative Adversarial Networks based on current understanding and research. As this is a rapidly evolving field, some information may become outdated over time. We encourage readers to consult recent academic papers and reputable sources for the most up-to-date information. If you notice any inaccuracies in this post, please report them so we can correct them promptly.