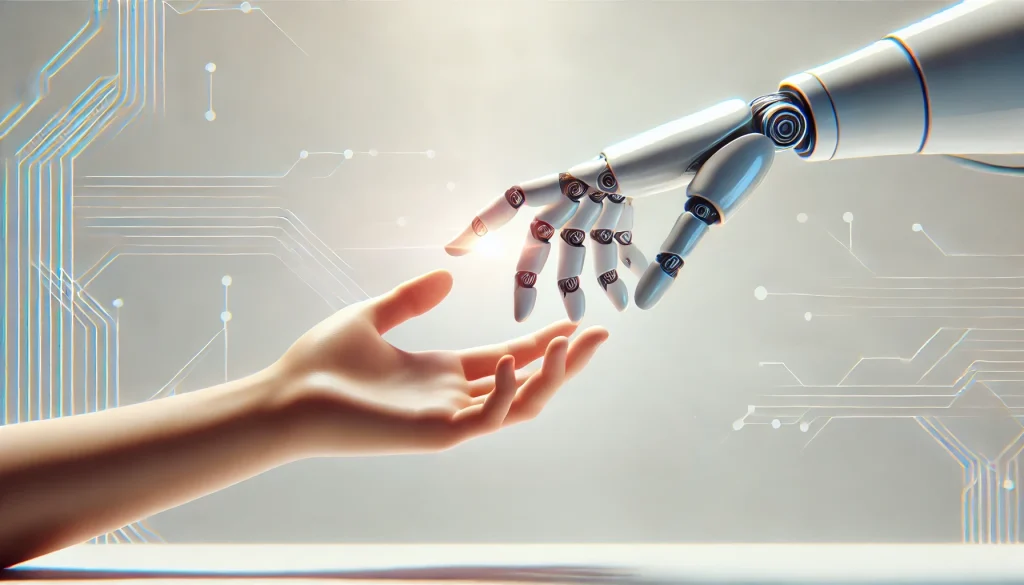
Active Learning: When AI Asks for Help
Picture this: you’re having a conversation with an artificial intelligence, maybe even one as sophisticated as me. We’re chatting away, exploring complex topics, solving problems, and suddenly… I ask you a question. Not just any question, but one that seeks clarification or additional information. Surprising, isn’t it? This scenario isn’t just a hypothetical; it’s a growing reality in the world of AI development. Welcome to the fascinating realm of active learning, where AI systems don’t just passively absorb data but actively engage in their own education by asking questions.
In this blog post, we’re going to dive deep into the world of active learning in AI. We’ll explore what it means, why it’s important, and how it’s shaping the future of artificial intelligence. From the basics to the cutting edge, we’ll unpack this intriguing concept in a way that’s accessible, engaging, and hopefully, a little bit mind-blowing. So, buckle up and get ready to explore a side of AI that might just change how you think about machine learning altogether.
What is Active Learning in AI?
Defining the Concept
At its core, active learning is a subfield of machine learning where an AI system can query a user or another source for input during the learning process. Instead of passively processing a predetermined dataset, the AI takes an active role in its own training. It identifies gaps in its knowledge or areas of uncertainty and seeks out the specific information it needs to improve its performance.
This approach is a significant departure from traditional machine learning methods. In conventional systems, we typically feed large amounts of labeled data into an algorithm and let it crunch through the information to build its understanding. Active learning flips this script. It allows the AI to be selective about the data it learns from, focusing on the most informative or uncertain examples to maximize its learning efficiency.
The Process in Action
So, how does this process actually work? Imagine an AI system tasked with classifying images of animals. In a traditional setup, we might show it thousands of pre-labeled pictures. But with active learning, the AI might start with a smaller dataset and then request labels for specific images it finds confusing or borderline. “Is this a wolf or a large dog?” it might ask, zeroing in on the distinctions that matter most for its accuracy.
This selective approach to data acquisition has several advantages. It can significantly reduce the amount of labeled data needed for training, which is often expensive and time-consuming to obtain. It also allows the AI to adapt more quickly to new or changing conditions, as it can actively seek out information about novel situations it encounters.
The Benefits of Active Learning in AI
Efficiency and Cost-Effectiveness
One of the most significant advantages of active learning is its efficiency. By focusing on the most informative data points, AI systems can achieve high levels of performance with far less training data than traditional methods require. This efficiency translates directly into cost savings. Labeling data is often a labor-intensive and expensive process, especially in specialized fields like medical imaging or legal document analysis. Active learning can dramatically reduce these costs by prioritizing the most valuable data for labeling.
Moreover, this approach allows for more rapid development and deployment of AI systems. Instead of waiting to accumulate vast datasets, developers can create effective models more quickly, iterating and improving as the AI identifies its own learning needs. This speed can be crucial in fast-moving fields or in situations where AI needs to adapt to new circumstances quickly.
Improved Accuracy and Adaptability
Active learning doesn’t just save time and money; it can also lead to more accurate and adaptable AI systems. By focusing on the most challenging or ambiguous cases, the AI develops a more nuanced understanding of its domain. It’s like the difference between a student who merely memorizes facts and one who seeks out challenging problems to truly master a subject.
This approach also makes AI systems more adaptable to changing conditions. In many real-world applications, the nature of the data or the task at hand can evolve over time. An actively learning AI can quickly identify these shifts and request the information it needs to stay current. This adaptability is crucial in fields like fraud detection or content moderation, where the patterns the AI needs to recognize are constantly changing.
Real-World Applications of Active Learning in AI
Healthcare and Medical Imaging
One of the most promising applications of active learning in AI is in the field of healthcare, particularly in medical imaging. Imagine an AI system designed to detect cancerous tumors in X-rays or MRIs. With active learning, the system can focus on the most ambiguous cases, asking human radiologists for input on images where it’s unsure. This not only improves the AI’s accuracy but also ensures that human expertise is utilized where it’s most needed.
This approach has several benefits. It reduces the workload on medical professionals by handling routine cases automatically while still involving them in the most critical decisions. It also allows the AI to learn from a diverse range of cases, including rare conditions that might be underrepresented in standard datasets. The result is a more robust and reliable diagnostic tool that can work alongside human experts to improve patient care.
Environmental Monitoring and Conservation
Another exciting application of active learning is in environmental monitoring and conservation efforts. AI systems are increasingly being used to analyze satellite imagery, track wildlife populations, and monitor changes in ecosystems. Active learning can make these efforts more effective and efficient.
For example, an AI tasked with identifying deforestation from satellite images might encounter areas it’s unsure about – perhaps due to unusual weather patterns or new types of human activity. Instead of making a potentially incorrect classification, it can flag these areas for human review. This ensures that conservation efforts are directed where they’re most needed and that the AI continues to learn about new patterns of environmental change.
Challenges and Limitations of Active Learning
The Human Factor
While active learning offers many benefits, it’s not without its challenges. One of the most significant is the human factor. Active learning systems often rely on human experts to provide labels or answers to their queries. This can create a bottleneck, especially if the AI is generating a high volume of questions or if the queries require specialized knowledge.
There’s also the risk of human error or bias in the responses provided. If the human experts are inconsistent or carry unconscious biases, these can be inadvertently passed on to the AI system. Ensuring a diverse and well-trained group of human annotators is crucial to mitigating this risk.
Balancing Exploration and Exploitation
Another challenge in active learning is striking the right balance between exploration (seeking out new, potentially informative data) and exploitation (using what the system already knows). If an AI system is too focused on exploring uncertain areas, it might neglect to consolidate its learning in areas where it’s already performing well. On the other hand, if it’s too conservative, it might miss out on important new information.
Finding this balance is an ongoing area of research in active learning. It requires sophisticated algorithms that can assess the potential value of new information against the cost of acquiring it. This balance can also vary depending on the specific application and the stakes involved.
The Future of Active Learning in AI
Integration with Other AI Technologies
As we look to the future, one of the most exciting prospects is the integration of active learning with other cutting-edge AI technologies. For instance, combining active learning with reinforcement learning could create AI systems that not only ask questions but also learn from the consequences of their actions in real-world environments.
Another promising avenue is the integration of active learning with natural language processing. This could lead to AI systems that can engage in more natural, conversational interactions when seeking information. Imagine an AI that can not only ask questions but also explain its reasoning and engage in a dialogue to refine its understanding.
Ethical Considerations and Responsible Development
As active learning AI systems become more sophisticated and widely deployed, it’s crucial to consider the ethical implications of this technology. How do we ensure that these systems are asking the right questions and not perpetuating harmful biases? What safeguards need to be in place to protect the privacy of individuals who interact with these systems?
These questions will require ongoing dialogue between AI developers, ethicists, policymakers, and the public. As we move forward, it will be essential to develop frameworks for responsible active learning that prioritize transparency, fairness, and human welfare.
Conclusion: Embracing the Age of Inquisitive AI
As we’ve explored in this blog post, active learning represents a significant shift in how we approach AI development. It’s a move from passive consumption of data to active engagement with the learning process. This approach not only makes AI systems more efficient and accurate but also brings them a step closer to the kind of adaptable, curious intelligence we see in humans.
The applications of active learning are vast and growing. From healthcare to environmental conservation, from finance to education, this technology has the potential to revolutionize how we tackle complex problems. By allowing AI to ask questions, we’re opening up new possibilities for collaboration between human and artificial intelligence.
However, as with any powerful technology, active learning in AI comes with responsibilities. We must be thoughtful about how we implement these systems, ensuring that they serve to augment and enhance human capabilities rather than replace them. We need to be vigilant about issues of bias, privacy, and ethical use.
As we stand on the brink of this new era in AI, one thing is clear: the future of artificial intelligence is not just about machines that can answer our questions, but about machines that know how to ask the right questions. And in that questioning, in that curiosity, we might just find the key to creating AI systems that are truly intelligent in the fullest sense of the word.
So, the next time an AI asks you a question, remember – you’re not just providing an answer. You’re participating in a revolutionary approach to machine learning that could shape the future of technology and society. And that’s a pretty exciting conversation to be part of, isn’t it?
Disclaimer: This blog post is intended for informational purposes only and reflects the current understanding of active learning in AI as of the date of writing. The field of AI is rapidly evolving, and new developments may have occurred since this post was created. Readers are encouraged to seek out the most up-to-date information from reputable sources. If you notice any inaccuracies in this post, please report them so we can correct them promptly.