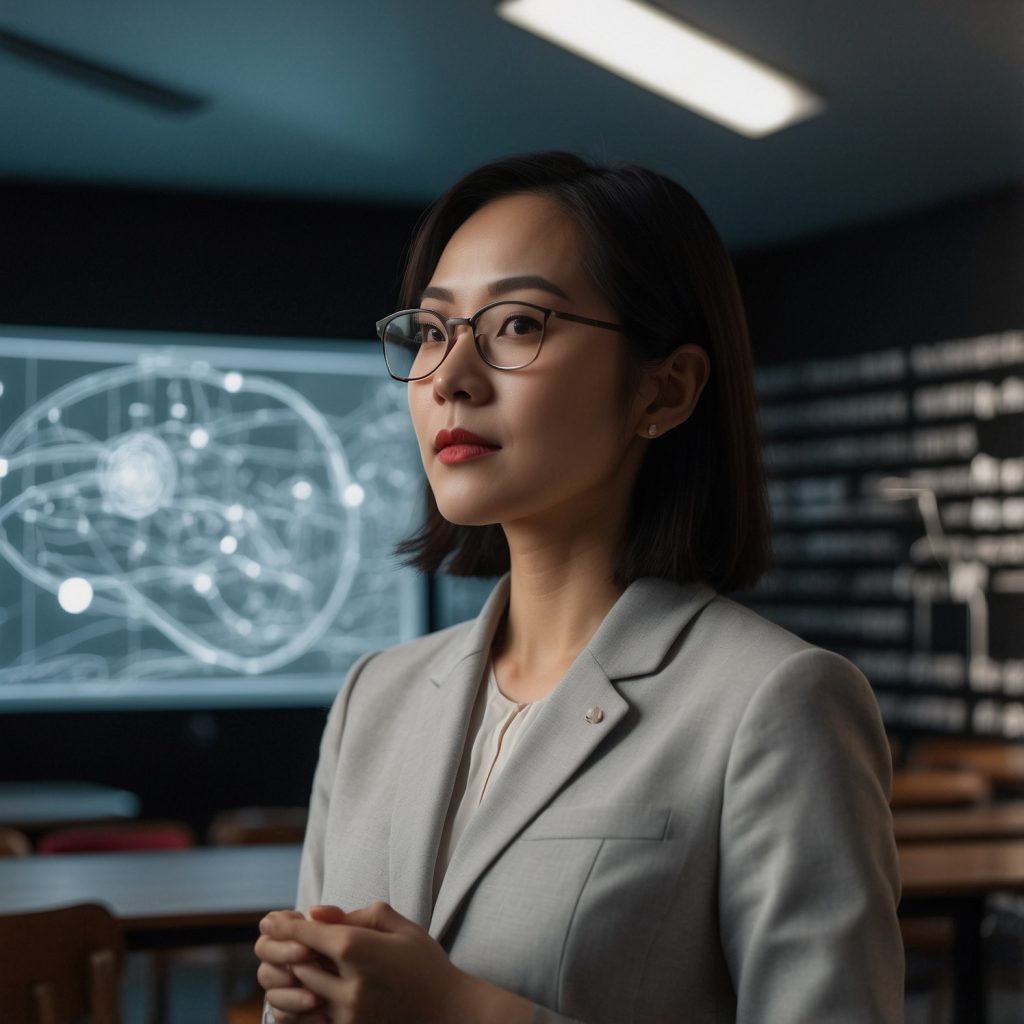
AI and Machine Learning: Understanding the Difference
Artificial Intelligence (AI) and Machine Learning (ML) are two terms that are often used interchangeably, but they are not the same. Both play a crucial role in the tech industry, powering innovations and shaping the future. However, understanding their differences is key to grasping their unique contributions and potential. In this blog, we will delve into what AI and ML are, how they differ, and why these distinctions matter. We will also explore their applications, benefits, and future trends.
What is Artificial Intelligence?
Defining AI: Artificial Intelligence refers to the simulation of human intelligence in machines that are programmed to think and learn like humans. AI can perform tasks that typically require human intelligence, such as recognizing speech, making decisions, and translating languages.
Types of AI: AI can be categorized into three types: Narrow AI, General AI, and Superintelligent AI. Narrow AI is designed to perform specific tasks, such as voice recognition systems like Siri or Alexa. General AI, which is still theoretical, would have the ability to perform any intellectual task that a human can. Superintelligent AI, a concept popularized by science fiction, refers to AI that surpasses human intelligence.
Components of AI: AI encompasses a variety of technologies, including machine learning, natural language processing, robotics, and computer vision. Each of these components contributes to making machines more intelligent and capable of performing complex tasks.
AI in Daily Life: AI has become an integral part of our daily lives. From personalized recommendations on streaming services to advanced fraud detection systems in banking, AI is used to improve efficiency and provide better user experiences.
Benefits of AI: The benefits of AI are vast. It can process large amounts of data quickly, identify patterns and trends, automate repetitive tasks, and improve decision-making processes. This leads to increased productivity and innovation across various industries.
Challenges of AI: Despite its advantages, AI also poses several challenges. These include ethical concerns, job displacement, privacy issues, and the need for significant computational resources. Addressing these challenges is crucial for the responsible development and deployment of AI technologies.
Future of AI: The future of AI is promising, with ongoing advancements in areas such as autonomous vehicles, healthcare diagnostics, and smart cities. As AI continues to evolve, it will play an even more significant role in shaping the future.
What is Machine Learning?
Defining ML: Machine Learning is a subset of AI that involves the development of algorithms that allow computers to learn from and make predictions or decisions based on data. ML focuses on building systems that can automatically improve and adapt over time with experience.
Types of ML: Machine Learning is generally divided into three types: Supervised Learning, Unsupervised Learning, and Reinforcement Learning. Supervised Learning involves training a model on labeled data, where the correct output is known. Unsupervised Learning deals with unlabeled data and tries to find hidden patterns or intrinsic structures. Reinforcement Learning is a type of learning where an agent learns to make decisions by taking actions in an environment to maximize some notion of cumulative reward.
Components of ML: Key components of machine learning include data collection, data preparation, model training, model evaluation, and deployment. Each of these steps is critical for building effective machine learning models.
ML in Daily Life: Machine learning powers many applications that we use daily. For example, spam filters in email, recommendation engines on e-commerce websites, and predictive text features on smartphones are all driven by ML algorithms.
Benefits of ML: Machine learning offers numerous benefits, such as automating complex tasks, improving accuracy in predictions, enhancing personalization in services, and driving innovation in fields like healthcare, finance, and transportation.
Challenges of ML: Machine learning also comes with its own set of challenges. These include the need for large amounts of high-quality data, the risk of overfitting or underfitting models, and the difficulty in interpreting complex models. Ensuring fairness and avoiding bias in ML models are also significant concerns.
Future of ML: The future of machine learning looks bright, with continuous advancements in areas like deep learning, natural language processing, and computer vision. As ML techniques become more sophisticated, their applications will expand further, driving progress in various domains.
Key Differences Between AI and ML
Scope: AI is a broader concept that encompasses the simulation of human intelligence by machines. ML, on the other hand, is a specific approach to achieving AI, focusing on the ability of machines to learn from data.
Objective: The primary objective of AI is to create systems that can perform tasks requiring human intelligence. The goal of ML is to develop algorithms that allow machines to learn from and make predictions based on data.
Approach: AI includes a wide range of techniques and technologies, including rule-based systems, expert systems, and machine learning. ML specifically involves developing and using algorithms that enable machines to learn from data.
Autonomy: AI systems can operate independently and make decisions without human intervention. ML models require training and input data but can operate autonomously once trained.
Applications: AI has broader applications, from robotics and autonomous systems to natural language processing and computer vision. ML is primarily used for tasks that involve pattern recognition, data analysis, and predictive modeling.
Complexity: AI systems can be highly complex and require the integration of various technologies. ML models can also be complex, but their complexity is often focused on the data and algorithms used.
Development: Developing AI systems often involves creating comprehensive architectures that integrate multiple technologies. Building ML models typically focuses on data collection, model selection, and training.
Applications of AI and ML
Healthcare: AI and ML are revolutionizing healthcare by improving diagnostics, personalizing treatment plans, and predicting patient outcomes. For example, AI-powered imaging systems can detect diseases like cancer at early stages, while ML algorithms can analyze patient data to suggest optimal treatments.
Finance: In the finance sector, AI and ML are used for fraud detection, risk assessment, and algorithmic trading. ML models can analyze transaction patterns to identify fraudulent activities, while AI systems can optimize trading strategies based on market data.
Retail: AI and ML enhance the retail experience by providing personalized recommendations, optimizing supply chains, and improving customer service. For instance, recommendation engines on e-commerce platforms suggest products based on user behavior, while AI-powered chatbots assist customers in real-time.
Transportation: AI and ML are driving innovation in transportation through the development of autonomous vehicles, traffic management systems, and predictive maintenance. Autonomous cars use AI to navigate and make decisions, while ML algorithms predict maintenance needs to prevent breakdowns.
Manufacturing: In manufacturing, AI and ML improve quality control, optimize production processes, and enhance supply chain management. AI-powered systems can detect defects in products, while ML models predict demand to optimize inventory levels.
Education: AI and ML are transforming education by personalizing learning experiences, automating administrative tasks, and providing insights into student performance. Adaptive learning platforms use AI to tailor content to individual students, while ML analyzes data to identify areas for improvement.
Benefits and Challenges of AI and ML
Efficiency: AI and ML enhance efficiency by automating repetitive tasks and processing large volumes of data quickly. This leads to significant time and cost savings for businesses.
Innovation: The ability of AI and ML to analyze data and identify patterns drives innovation across various industries. These technologies enable the development of new products, services, and business models.
Decision Making: AI and ML improve decision-making processes by providing data-driven insights and predictions. This helps organizations make informed decisions and respond to changing market conditions effectively.
Accuracy: AI and ML enhance accuracy in tasks such as data analysis, image recognition, and natural language processing. This reduces errors and improves the quality of outcomes.
Job Creation: While there are concerns about job displacement, AI and ML also create new job opportunities in fields such as data science, AI ethics, and machine learning engineering.
Ethical Concerns: The development and deployment of AI and ML raise ethical concerns, including issues related to privacy, bias, and accountability. Ensuring ethical use of these technologies is crucial for their sustainable growth.
Resource Intensive: AI and ML require significant computational resources and large datasets for training models. This can be a barrier for smaller organizations and individuals.
Interpretability: Understanding and interpreting complex AI and ML models can be challenging. This is especially important in critical applications where transparency is necessary.
Future Trends in AI and ML
Advancements in Deep Learning: Deep learning, a subset of ML, is expected to drive significant advancements in AI. Techniques such as neural networks and reinforcement learning will lead to more sophisticated and capable AI systems.
AI in Edge Computing: AI is moving towards edge computing, where data processing occurs closer to the source rather than in centralized data centers. This reduces latency and improves the efficiency of AI applications in real-time environments.
Explainable AI: As AI systems become more complex, the need for explainable AI grows. Researchers are developing methods to make AI models more transparent and interpretable, ensuring that their decisions can be understood and trusted.
AI Ethics: The ethical implications of AI are gaining more attention. Efforts are being made to develop guidelines and frameworks to ensure the responsible and fair use of AI technologies.
AI in Healthcare: AI’s role in healthcare will continue to expand, with advancements in precision medicine, drug discovery, and personalized treatment plans. AI-powered diagnostic tools will become more accurate and widely adopted.
AI and Sustainability: AI is being leveraged to address environmental challenges and promote sustainability. Applications include optimizing energy consumption, predicting climate patterns, and improving resource management.
Human-AI Collaboration: The future will see increased collaboration between humans and AI systems. AI will augment human capabilities, enabling more effective problem-solving and decision-making.
AI in Cybersecurity: AI will play a critical role in enhancing cybersecurity by detecting and responding to threats in real-time. ML algorithms will analyze patterns to identify potential security breaches and vulnerabilities.
Conclusion
Understanding the difference between AI and ML is essential for appreciating their unique contributions and potential. While AI is the broader concept of creating intelligent machines, ML is a subset that focuses on enabling these machines to learn from data. Both technologies have transformative applications across various industries, enhancing efficiency, innovation, and decision-making processes.
As AI and ML continue to evolve, their impact will grow, driving advancements in fields like healthcare, finance, transportation, and education. However, with these advancements come challenges such as ethical concerns, resource requirements, and the need for interpretability. Addressing these challenges is crucial for the responsible and sustainable development of AI and ML technologies.
The future of AI and ML is promising, with trends pointing towards deeper integration into our daily lives, enhanced collaboration between humans and machines, and significant contributions to solving global challenges. By understanding their differences and harnessing their potential responsibly, we can look forward to a future where AI and ML not only enhance our capabilities but also contribute to a better, more efficient world.
In conclusion, AI and ML are at the forefront of technological innovation. Their differences highlight the diverse approaches and applications that drive their development. Embracing both technologies while addressing their challenges will pave the way for a future where intelligent systems enhance our lives and open up new possibilities.