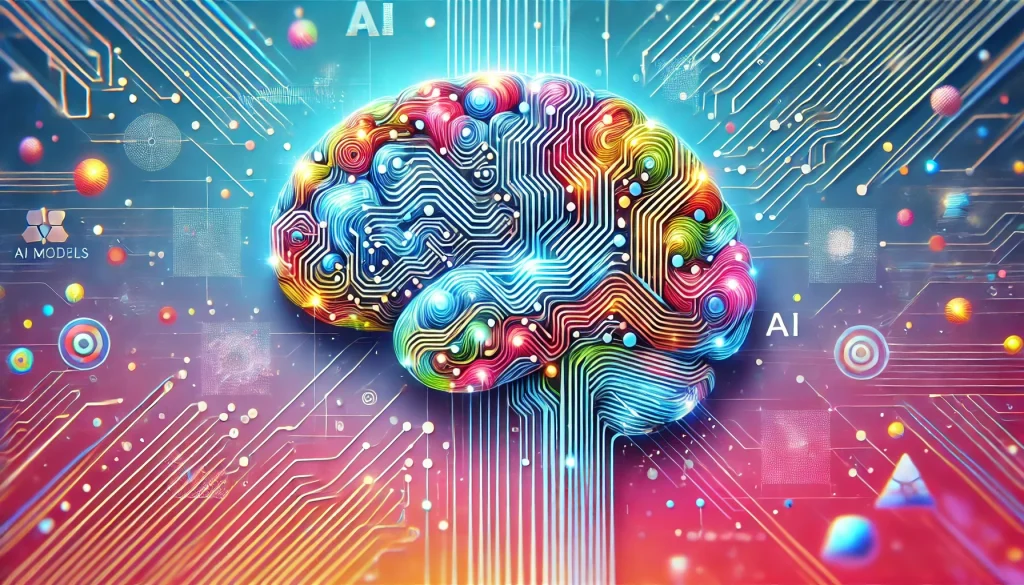
AI Models: The Brains Behind Intelligent Systems
Artificial Intelligence (AI) is not just a buzzword; it’s the heartbeat of our digital era. From Siri reminding you of your next appointment to self-driving cars cruising the streets, AI models are the unsung heroes making it all happen. But what exactly are these AI models? How do they function? Why are they so crucial in today’s tech-driven world? Let’s dive deep into the fascinating realm of AI models, the true brains behind intelligent systems.
The Genesis of AI Models
Understanding the Origins: The journey of AI models began decades ago. It all started with the dream of creating machines that could mimic human intelligence. Early pioneers like Alan Turing and John McCarthy laid the groundwork for what we now call AI. They envisaged machines that could think, learn, and adapt just like humans. The development of AI models was fueled by this vision, propelling the technology forward.
Early Days and Evolution: Initially, AI models were rudimentary, focusing on simple problem-solving tasks. These early models, known as rule-based systems, relied heavily on predefined rules and logic. While they could handle straightforward tasks, their limitations became evident as tasks grew more complex. This led to the development of more sophisticated models, capable of learning and evolving on their own.
The Role of Data: Data is the lifeblood of AI models. Without data, these models cannot learn or improve. In the early days, obtaining and processing data was a significant challenge. However, with the advent of the internet and the proliferation of digital devices, data became more accessible. This data explosion has been a catalyst for the rapid advancement of AI models.
Types of AI Models
Supervised Learning Models: One of the most common types of AI models is supervised learning. These models are trained using labeled data, meaning the input and the corresponding output are known. Supervised learning is like having a teacher guiding the model, correcting its mistakes, and helping it learn from them. Examples include image recognition, where a model is trained to identify objects in images, and language translation, where it learns to translate text from one language to another.
Unsupervised Learning Models: Unlike supervised learning, unsupervised learning models work with unlabeled data. These models try to identify patterns and relationships within the data without any guidance. It’s akin to exploring a new city without a map, relying on intuition to find connections. Clustering and dimensionality reduction are common techniques in unsupervised learning. These models are particularly useful in scenarios where labeling data is impractical or impossible.
Reinforcement Learning Models: Inspired by behavioral psychology, reinforcement learning models learn by interacting with their environment. They make decisions, receive feedback in the form of rewards or penalties, and adjust their actions accordingly. It’s like training a dog; good behavior is rewarded, encouraging the dog to repeat it. Reinforcement learning is widely used in robotics, gaming, and autonomous vehicles, where the model needs to adapt to dynamic environments.
Deep Learning Models: A subset of machine learning, deep learning models are designed to mimic the human brain’s neural networks. These models consist of multiple layers, each processing the input data and passing it to the next layer. Deep learning has revolutionized fields like computer vision and natural language processing, enabling breakthroughs such as facial recognition and voice assistants. The complexity and depth of these models allow them to handle intricate tasks with remarkable accuracy.
How AI Models Work
The Training Process: Training an AI model is a meticulous process. It begins with collecting and preparing data. This data is then split into training and validation sets. The model is trained using the training set, learning to recognize patterns and make predictions. The validation set is used to evaluate the model’s performance and fine-tune its parameters. This iterative process continues until the model achieves the desired accuracy.
Feature Extraction and Engineering: Feature extraction is a critical step in building AI models. It involves identifying the most relevant attributes or features from the data that will help the model make accurate predictions. Feature engineering goes a step further, creating new features from the existing ones to enhance the model’s performance. This step requires domain expertise and creativity, as the quality of the features significantly impacts the model’s effectiveness.
Model Selection and Evaluation: Choosing the right model is crucial. Different tasks require different models, and selecting the most appropriate one can make a significant difference. Once a model is chosen, it’s rigorously evaluated using metrics like accuracy, precision, recall, and F1 score. These metrics provide insights into the model’s performance, highlighting areas that need improvement.
Deployment and Monitoring: After training and evaluation, the model is deployed in a real-world environment. However, the journey doesn’t end here. Continuous monitoring is essential to ensure the model remains effective over time. Changes in data patterns or external factors can impact the model’s performance, necessitating periodic retraining and updates.
Applications of AI Models
Healthcare: AI models are transforming healthcare by enabling early diagnosis and personalized treatment plans. For instance, models trained on medical images can detect diseases like cancer with high accuracy, often surpassing human experts. Predictive models analyze patient data to forecast potential health issues, allowing for proactive intervention.
Finance: In the finance sector, AI models are used for fraud detection, risk assessment, and algorithmic trading. They analyze vast amounts of transaction data to identify suspicious activities, protecting both institutions and customers. Additionally, AI-driven financial advisors provide personalized investment recommendations, optimizing portfolio performance.
Retail: AI models enhance the retail experience by personalizing customer interactions and optimizing inventory management. Recommendation systems suggest products based on customer preferences, increasing sales and customer satisfaction. Inventory management models predict demand, ensuring that stock levels are maintained efficiently.
Transportation: Autonomous vehicles are a prime example of AI models in transportation. These vehicles use a combination of supervised, unsupervised, and reinforcement learning models to navigate roads safely. Predictive models also optimize logistics and supply chain operations, reducing costs and improving delivery times.
Entertainment: In the entertainment industry, AI models create personalized content recommendations, enhancing user engagement. Streaming services like Netflix and Spotify use these models to suggest movies, TV shows, and music based on individual preferences. AI is also used in content creation, generating music, artwork, and even scripts for movies.
Challenges and Future Directions
Data Privacy and Security: One of the biggest challenges in AI is ensuring data privacy and security. As models rely on vast amounts of data, safeguarding this data from breaches and misuse is paramount. Striking a balance between data utility and privacy is an ongoing challenge that researchers and policymakers are grappling with.
Bias and Fairness: AI models are only as good as the data they are trained on. Biased data can lead to biased models, perpetuating existing inequalities. Ensuring fairness and inclusivity in AI models is crucial. Researchers are developing techniques to detect and mitigate bias, promoting more equitable outcomes.
Explainability and Transparency: Many AI models, especially deep learning models, are often considered “black boxes” due to their complexity. Understanding how these models make decisions is challenging but essential for trust and accountability. Efforts are underway to develop explainable AI, providing insights into the decision-making process of these models.
Ethical Considerations: The ethical implications of AI are vast. From job displacement due to automation to the use of AI in surveillance, these technologies raise significant ethical questions. Ensuring that AI is developed and deployed responsibly, with a focus on societal benefits, is critical.
Advancements in AI Research: The future of AI is promising, with ongoing research pushing the boundaries of what is possible. Innovations in areas like quantum computing, neuromorphic engineering, and cognitive architectures are set to revolutionize AI models. These advancements will enable even more sophisticated and intelligent systems, transforming industries and our daily lives.
Conclusion
AI models are the brains behind the intelligent systems that are becoming an integral part of our lives. From healthcare to entertainment, these models are driving innovation and enhancing our experiences. While challenges like data privacy, bias, and ethics need to be addressed, the potential of AI models is immense. As research continues to advance, we can look forward to even more groundbreaking applications that will shape the future.
Disclaimer: This blog is intended for informational purposes only. The views and opinions expressed in this blog are those of the author and do not necessarily reflect the official policy or position of any agency or organization. Please report any inaccuracies so we can correct them promptly.