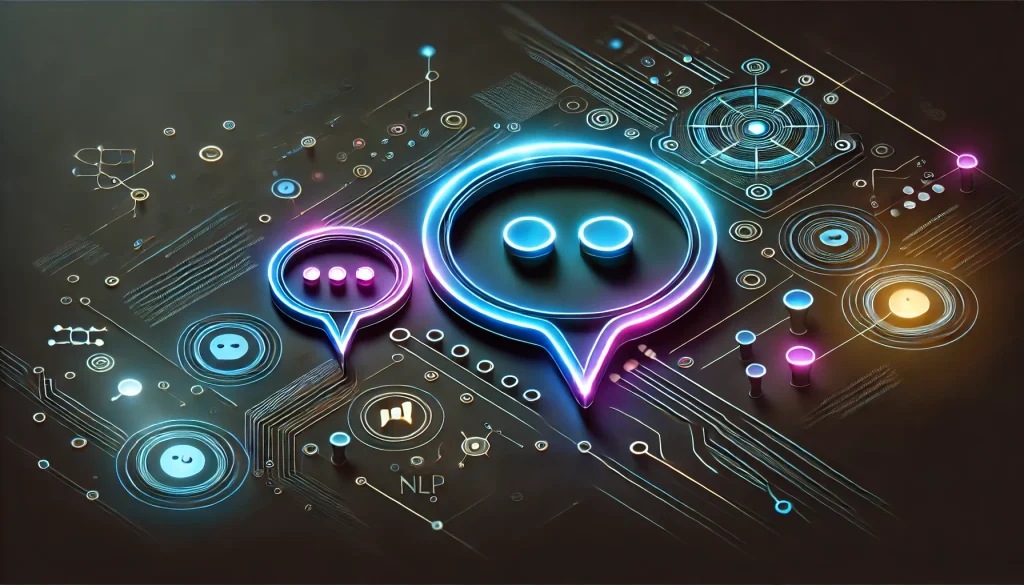
Discourse Analysis in NLP: Understanding Conversations with AI
Natural Language Processing (NLP) has revolutionized how we interact with technology, and discourse analysis is a key aspect of this field. By understanding conversations and context, AI can provide more accurate and meaningful interactions. This blog explores the fascinating world of discourse analysis in NLP, its applications, and its impact on our daily lives.
What is Discourse Analysis in NLP?
Understanding the Basics
Discourse analysis involves studying written or spoken language in context. It’s about understanding how sentences are connected and how meaning is constructed in communication. In NLP, this means teaching AI to not only understand individual words and sentences but also grasp the bigger picture of a conversation. For instance, when we say, “The weather is terrible today,” followed by, “I don’t want to go out,” discourse analysis helps the AI understand that the second sentence is influenced by the first.
The Importance of Context
Context is king in discourse analysis. A sentence can have different meanings depending on its context. For example, the phrase “It’s cold” can refer to the temperature of the room, someone’s attitude, or even a drink. Discourse analysis in NLP aims to teach AI to discern these subtleties by analyzing surrounding text and recognizing patterns in communication.
Components of Discourse Analysis
There are several key components in discourse analysis:
- Cohesion: How different parts of a text are connected. This includes the use of pronouns, conjunctions, and other linking words.
- Coherence: The overall sense and logical flow of a conversation.
- Pragmatics: The study of how context influences meaning.
- Speech Acts: Actions performed via utterances, like making requests, promises, or apologies.
The Role of AI in Discourse Analysis
Advancements in AI Technology
AI technology has come a long way in understanding human language. Machine learning algorithms, deep learning models, and vast datasets have enabled AI to analyze and interpret language with increasing accuracy. Techniques like sentiment analysis, topic modeling, and named entity recognition are all part of this advancement, but discourse analysis takes it a step further.
Machine Learning and Deep Learning
Machine learning algorithms are trained on large datasets to recognize patterns in language. Deep learning models, particularly those based on neural networks, have shown remarkable success in understanding context and generating coherent responses. These models learn from vast amounts of text data, identifying relationships between words, sentences, and larger discourse structures.
Applications of Discourse Analysis in NLP
Discourse analysis is used in various NLP applications:
- Chatbots and Virtual Assistants: Enhancing their ability to understand and respond to user queries contextually.
- Sentiment Analysis: Understanding the tone and emotional context of a conversation.
- Summarization: Creating concise summaries of long texts while preserving the main ideas.
- Translation: Improving the accuracy of translations by considering the context of the entire text.
Practical Applications of Discourse Analysis
Chatbots and Virtual Assistants
One of the most visible applications of discourse analysis in NLP is in chatbots and virtual assistants like Siri, Alexa, and Google Assistant. These AI-driven systems rely on discourse analysis to understand user queries and provide relevant responses. For instance, if you ask your virtual assistant about the weather and then follow up with a question about outdoor activities, the assistant should understand the connection between the two questions.
Improving Customer Service
In customer service, discourse analysis helps chatbots handle complex interactions more effectively. By understanding the flow of a conversation, these bots can provide better support, answer follow-up questions, and even detect when a human intervention is needed. This not only improves customer satisfaction but also reduces the workload on human agents.
Sentiment Analysis and Opinion Mining
Discourse analysis plays a crucial role in sentiment analysis and opinion mining. By understanding the context of user reviews, social media posts, and other textual data, AI can accurately gauge the sentiment behind the words. This is invaluable for businesses looking to understand customer opinions and improve their products and services.
Content Summarization
Automatic summarization of long documents, articles, and reports is another area where discourse analysis is essential. By identifying the main points and ignoring irrelevant details, AI can generate concise summaries that capture the essence of the original text. This is particularly useful in fields like journalism, law, and academia.
Enhancing Translation Services
Translation services benefit greatly from discourse analysis. Understanding the context of a conversation or a document ensures more accurate translations. This is especially important for languages with complex grammatical structures or where context significantly changes the meaning of words.
Challenges in Discourse Analysis
Ambiguity in Language
One of the biggest challenges in discourse analysis is dealing with ambiguity. Words and sentences can have multiple meanings, and context is crucial to disambiguating them. For example, the word “bank” can refer to a financial institution or the side of a river. AI must be able to use surrounding text to determine the correct meaning.
Cultural and Contextual Differences
Language is deeply influenced by culture and context. Idioms, slang, and cultural references can be difficult for AI to understand without extensive training on diverse datasets. For instance, the phrase “break a leg” means good luck in English, but taken literally, it has a completely different meaning.
Sarcasm and Irony
Detecting sarcasm and irony is another significant challenge. These forms of expression rely heavily on tone and context, which can be difficult for AI to interpret. For example, the sentence “Great, just what I needed” can be sincere or sarcastic depending on the situation.
Future Directions in Discourse Analysis
Advancements in Deep Learning
Future advancements in deep learning and neural networks hold promise for overcoming many of the challenges in discourse analysis. More sophisticated models, such as transformers and attention mechanisms, are already showing improvements in understanding context and generating coherent responses.
Multimodal Analysis
Combining text analysis with other forms of data, such as images, audio, and video, can provide richer context and improve AI’s understanding of conversations. For instance, analyzing facial expressions and voice tone alongside text can help detect sarcasm and emotional nuances.
Personalized AI
Personalized AI that adapts to individual users’ language patterns and preferences can enhance discourse analysis. By learning from specific user interactions, AI can provide more relevant and accurate responses. This personalization is particularly important in customer service and virtual assistants.
Ethical Considerations
As AI becomes more adept at understanding and generating human language, ethical considerations become increasingly important. Ensuring privacy, avoiding biases, and maintaining transparency are crucial to building trust in AI systems. Researchers and developers must prioritize these ethical concerns to create responsible AI technologies.
Conclusion
Discourse analysis in NLP is a fascinating and complex field that holds immense potential for improving AI’s understanding of human language. By grasping the nuances of context, coherence, and pragmatics, AI can engage in more meaningful and accurate conversations. From chatbots and virtual assistants to sentiment analysis and translation services, the applications are vast and impactful. As technology continues to advance, the challenges of ambiguity, cultural differences, and detecting sarcasm will be addressed, leading to even more sophisticated AI systems. The future of discourse analysis in NLP is bright, promising more seamless and intuitive interactions between humans and machines.
Disclaimer: The information provided in this blog is for educational purposes only. We strive for accuracy but acknowledge that errors may occur. Please report any inaccuracies so we can correct them promptly.