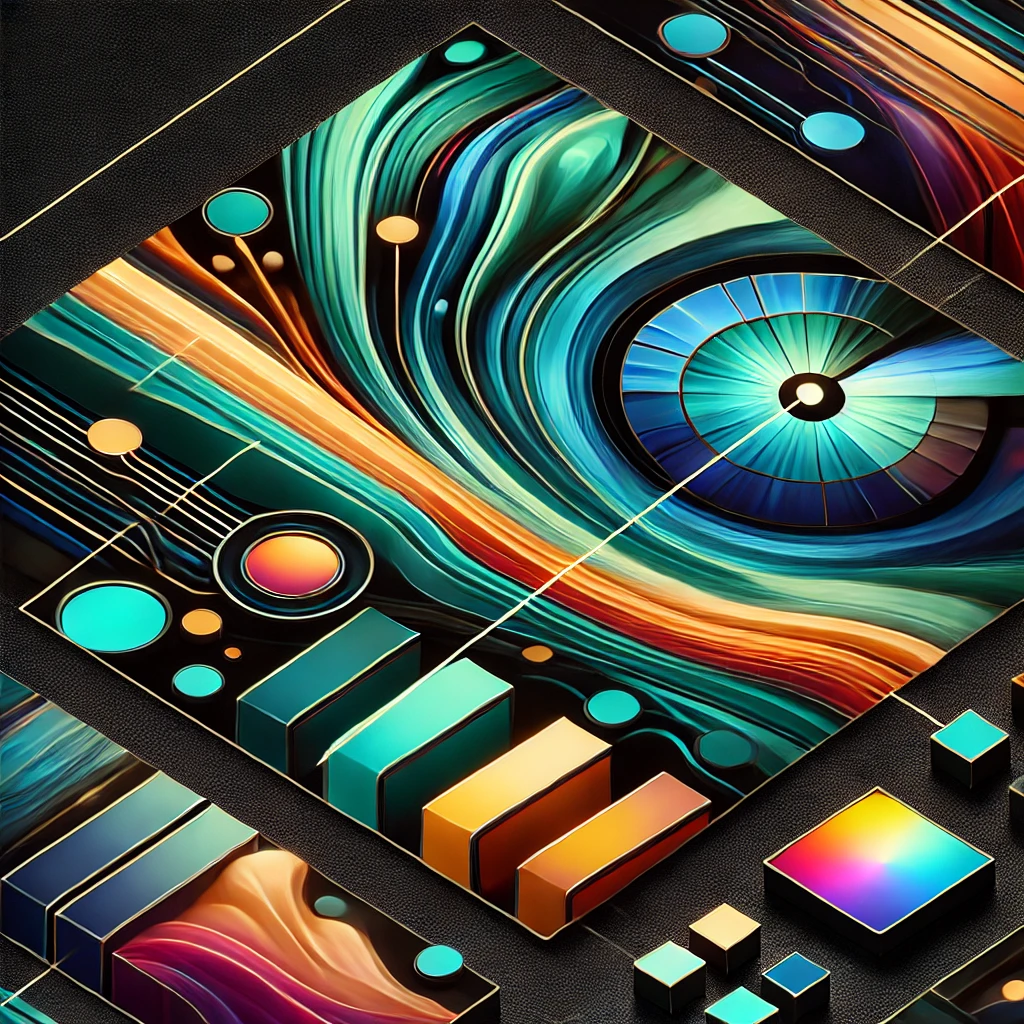
Image Segmentation: Dividing Images into Meaningful Parts with AI
Have you ever wondered how self-driving cars recognize pedestrians, or how medical imaging systems detect tumors? The answer lies in a fascinating field of artificial intelligence called image segmentation. This powerful technique is revolutionizing the way computers understand and interpret visual information, opening up a world of exciting possibilities across various industries. In this blog post, we’ll dive deep into the world of image segmentation, exploring its inner workings, applications, and the incredible impact it’s having on our daily lives. So, buckle up and get ready for a journey through the pixels!
What is Image Segmentation?
Let’s start with the basics. Image segmentation is a process that divides an image into multiple segments or regions, each representing a distinct object or part of the image. It’s like giving a computer the ability to “see” and understand the different components that make up a picture, much like how our brains naturally process visual information. But why is this so important? Well, by breaking down an image into meaningful parts, we enable machines to analyze and interpret visual data with incredible accuracy and efficiency.
The goal of image segmentation
The primary objective of image segmentation is to simplify the representation of an image, making it easier for computers to analyze and understand. By grouping pixels with similar characteristics, we can identify objects, boundaries, and regions of interest within an image. This process is crucial for a wide range of applications, from medical imaging and autonomous vehicles to facial recognition and even augmented reality. Imagine trying to find Waldo in a crowded scene – that’s essentially what image segmentation does, but for every object in the picture!
How does it differ from image classification?
While image segmentation and image classification might sound similar, they serve different purposes. Image classification assigns a single label to an entire image, answering the question, “What is this image of?” On the other hand, image segmentation goes a step further by identifying and localizing multiple objects within a single image. It’s like the difference between saying, “This is a picture of a living room,” and “This living room contains a sofa, two chairs, a coffee table, and a potted plant.” Segmentation provides a much more detailed understanding of the image content, making it invaluable for tasks that require precise object localization and boundary detection.
The Magic Behind Image Segmentation: How Does it Work?
Now that we understand what image segmentation is and why it’s important, let’s peek under the hood and explore how this fascinating technology actually works. At its core, image segmentation relies on sophisticated algorithms and machine learning techniques to analyze pixel patterns, colors, textures, and other visual features. These algorithms use this information to group similar pixels together and separate them from dissimilar ones, effectively “cutting” the image into meaningful segments.
Traditional approaches vs. deep learning
In the early days of image segmentation, researchers relied on traditional computer vision techniques such as thresholding, edge detection, and region growing. These methods worked well for simple images but often struggled with complex scenes and variations in lighting or texture. Enter deep learning – a game-changer in the field of artificial intelligence. With the advent of powerful neural networks, particularly Convolutional Neural Networks (CNNs), image segmentation has taken a giant leap forward. These advanced models can learn to recognize intricate patterns and features in images, leading to much more accurate and robust segmentation results.
Types of image segmentation
There are several types of image segmentation, each suited for different applications and challenges. Semantic segmentation assigns a class label to each pixel in the image, effectively coloring the entire image based on object categories. Instance segmentation goes a step further by distinguishing between individual instances of the same object class – for example, separating each car in a traffic scene. Panoptic segmentation combines both semantic and instance segmentation, providing a comprehensive understanding of the image. These different approaches allow researchers and developers to choose the most appropriate method for their specific needs, whether it’s analyzing medical scans or developing advanced driver assistance systems.
Real-World Applications: Image Segmentation in Action
The beauty of image segmentation lies in its versatility and wide-ranging applications. This powerful technique is making waves across various industries, transforming the way we interact with technology and solve complex problems. Let’s explore some of the most exciting and impactful applications of image segmentation in the real world.
Medical imaging and diagnostics
One of the most critical applications of image segmentation is in the field of medical imaging. By accurately segmenting medical scans such as MRIs, CT scans, and X-rays, AI can help doctors identify and analyze tumors, measure organ volumes, and detect abnormalities with unprecedented precision. This technology is not only improving diagnostic accuracy but also enabling personalized treatment plans and more effective patient monitoring. Imagine a world where early detection of diseases becomes the norm, thanks to AI-powered image segmentation – we’re already on our way there!
Autonomous vehicles and computer vision
Self-driving cars are no longer a distant dream, and image segmentation plays a crucial role in making them a reality. These vehicles rely on advanced computer vision systems to understand their surroundings, detect obstacles, and make split-second decisions. Image segmentation allows the car’s AI to identify and track pedestrians, other vehicles, road signs, and lane markings in real-time. This level of detailed understanding is essential for safe and efficient autonomous navigation. The next time you see a self-driving car smoothly navigating through traffic, remember that image segmentation is working behind the scenes to make it all possible.
Facial recognition and biometrics
From unlocking your smartphone to enhancing security systems, facial recognition has become an integral part of our daily lives. Image segmentation is a key component of these systems, allowing for precise identification of facial features and landmarks. By accurately segmenting different parts of the face, such as eyes, nose, and mouth, AI can create detailed facial maps that are unique to each individual. This technology extends beyond just faces – image segmentation is also used in fingerprint recognition, iris scanning, and other biometric applications, revolutionizing the way we approach security and authentication.
Augmented reality and virtual try-ons
Ever wondered how those fun Snapchat filters work, or how you can virtually try on glasses before buying them online? You guessed it – image segmentation is the secret sauce! By precisely segmenting facial features or body parts, AR applications can seamlessly overlay virtual elements onto the real world. This technology is not only entertaining but also has practical applications in industries like fashion, cosmetics, and interior design. Imagine redecorating your entire home virtually before making any purchases, or trying on dozens of outfits in seconds without ever stepping into a changing room – that’s the power of image segmentation in AR.
Challenges and Limitations: The Road Ahead
While image segmentation has come a long way and achieved remarkable results, it’s not without its challenges. As with any cutting-edge technology, there are still hurdles to overcome and limitations to address. Understanding these challenges is crucial for both developers working on improving the technology and users relying on image segmentation in their applications.
Dealing with complex scenes and occlusions
One of the biggest challenges in image segmentation is handling complex scenes with multiple overlapping objects or partial occlusions. Think of a crowded street scene or a busy kitchen – even humans can sometimes struggle to distinguish individual objects in such environments. For AI, this task is even more daunting. Current segmentation algorithms can sometimes struggle to accurately separate objects that are partially hidden or tightly packed together. Researchers are constantly working on developing more sophisticated models that can better handle these complex scenarios, drawing inspiration from how the human visual system processes such information.
Adapting to variations in lighting and perspective
Another significant challenge is creating segmentation models that can perform consistently across different lighting conditions and viewing angles. An object’s appearance can change dramatically depending on the time of day, weather conditions, or the angle from which it’s viewed. While humans can easily adapt to these variations, AI systems need to be explicitly trained to handle them. This often requires large and diverse datasets that capture objects under various conditions. Techniques like data augmentation and domain adaptation are being explored to make segmentation models more robust and versatile in real-world scenarios.
Balancing accuracy and computational efficiency
As image segmentation finds its way into more real-time applications, such as autonomous vehicles and augmented reality, there’s a growing need for algorithms that are both accurate and computationally efficient. High-quality segmentation often requires complex models that can be computationally intensive, potentially leading to latency issues in time-sensitive applications. Striking the right balance between segmentation accuracy and processing speed is an ongoing challenge. Researchers are exploring techniques like model compression, hardware acceleration, and edge computing to make image segmentation more viable for real-time and mobile applications.
The Future of Image Segmentation: What’s on the Horizon?
As we look to the future, the potential for image segmentation seems boundless. With rapid advancements in AI and computing power, we’re on the cusp of even more exciting developments in this field. Let’s explore some of the trends and innovations that are shaping the future of image segmentation.
3D and volumetric segmentation
While most current image segmentation techniques focus on 2D images, there’s a growing interest in 3D and volumetric segmentation. This approach is particularly valuable in medical imaging, where it can provide a more comprehensive understanding of anatomical structures. Imagine being able to segment and analyze entire organs or tumor volumes in three dimensions – this could revolutionize diagnosis and treatment planning. Beyond medicine, 3D segmentation has applications in fields like robotics, virtual reality, and autonomous navigation, where understanding the spatial relationships between objects is crucial.
Real-time segmentation on edge devices
As mobile devices and IoT sensors become more powerful, there’s a push to perform image segmentation directly on these edge devices rather than relying on cloud processing. This trend towards edge computing has several advantages, including reduced latency, improved privacy, and the ability to operate in areas with limited connectivity. Researchers are developing lightweight segmentation models and optimizing algorithms to run efficiently on mobile processors. In the near future, we might see smartphones capable of performing complex segmentation tasks in real-time, enabling a new generation of AI-powered mobile applications.
Multimodal segmentation
Another exciting direction is the integration of multiple data modalities for more accurate and comprehensive segmentation. Instead of relying solely on visual information, future segmentation systems might combine data from various sensors, such as depth cameras, infrared sensors, or even audio inputs. This multimodal approach could lead to more robust and context-aware segmentation, particularly in challenging environments. For instance, an autonomous vehicle might use a combination of visual, LiDAR, and radar data to achieve more reliable object segmentation in adverse weather conditions.
Self-supervised and few-shot learning
One of the main limitations of current segmentation models is their reliance on large, manually labeled datasets for training. To address this, researchers are exploring self-supervised and few-shot learning techniques. Self-supervised learning allows models to learn useful representations from unlabeled data, reducing the need for extensive manual annotation. Few-shot learning, on the other hand, aims to create models that can quickly adapt to new segmentation tasks with minimal labeled examples. These approaches could make it easier to deploy image segmentation in new domains and for rare or previously unseen object classes.
Ethical Considerations and Responsible Development
As image segmentation technology continues to advance and permeate various aspects of our lives, it’s crucial to consider the ethical implications and ensure responsible development. Like any powerful AI technology, image segmentation has the potential for both positive and negative impacts on society.
Privacy concerns
One of the primary ethical considerations surrounding image segmentation is privacy. As this technology becomes more accurate and ubiquitous, there are concerns about its potential misuse for surveillance or unauthorized data collection. For instance, advanced facial segmentation could be used to track individuals across multiple cameras or identify people in crowds without their consent. It’s essential for developers and policymakers to establish clear guidelines and regulations governing the use of image segmentation technology, particularly in public spaces and sensitive environments.
Bias and fairness
Another critical issue is the potential for bias in image segmentation models. If these models are trained on datasets that are not diverse or representative, they may perform poorly on certain demographic groups or in specific contexts. This could lead to unfair or discriminatory outcomes, especially in high-stakes applications like medical diagnosis or autonomous vehicle perception. Ensuring fairness and inclusivity in image segmentation requires careful consideration of dataset collection, model evaluation, and ongoing monitoring for bias.
Transparency and explainability
As image segmentation systems become more complex and are used in critical decision-making processes, there’s a growing need for transparency and explainability. Users and stakeholders should be able to understand how these systems arrive at their segmentation results, especially in applications where the consequences of errors could be significant. Developing interpretable models and creating tools for visualizing and explaining segmentation decisions are important areas of research that can help build trust and accountability in AI systems.
Conclusion
Image segmentation has come a long way from its humble beginnings, evolving into a powerful and versatile tool that’s reshaping how machines perceive and interact with the visual world. From saving lives through advanced medical diagnostics to enabling the next generation of augmented reality experiences, this technology is leaving an indelible mark on numerous industries and aspects of our daily lives.
As we’ve explored in this blog post, the journey of image segmentation is far from over. With ongoing research addressing current challenges and pushing the boundaries of what’s possible, we can expect even more exciting developments in the coming years. The integration of 3D segmentation, edge computing, and multimodal approaches promises to unlock new applications and improve existing ones.
However, as we march forward on this pixelated path, it’s crucial to remain mindful of the ethical considerations and potential societal impacts of this technology. By fostering responsible development, addressing privacy concerns, and striving for fairness and transparency, we can ensure that image segmentation continues to be a force for positive change.
Whether you’re a developer working on the cutting edge of AI, a business leader exploring how to leverage this technology, or simply a curious individual fascinated by the possibilities, the world of image segmentation offers a wealth of opportunities to explore and innovate. So, the next time you unlock your phone with your face or see a self-driving car smoothly navigate traffic, take a moment to appreciate the intricate dance of pixels and algorithms that make it all possible. The future of image segmentation is bright, and we’re just beginning to scratch the surface of its true potential.
Disclaimer: This blog post provides an overview of image segmentation technology based on current knowledge and developments. As this field is rapidly evolving, some information may become outdated over time. We encourage readers to consult the latest research and official sources for the most up-to-date information on image segmentation techniques and applications. If you notice any inaccuracies in this post, please report them so we can promptly make corrections.