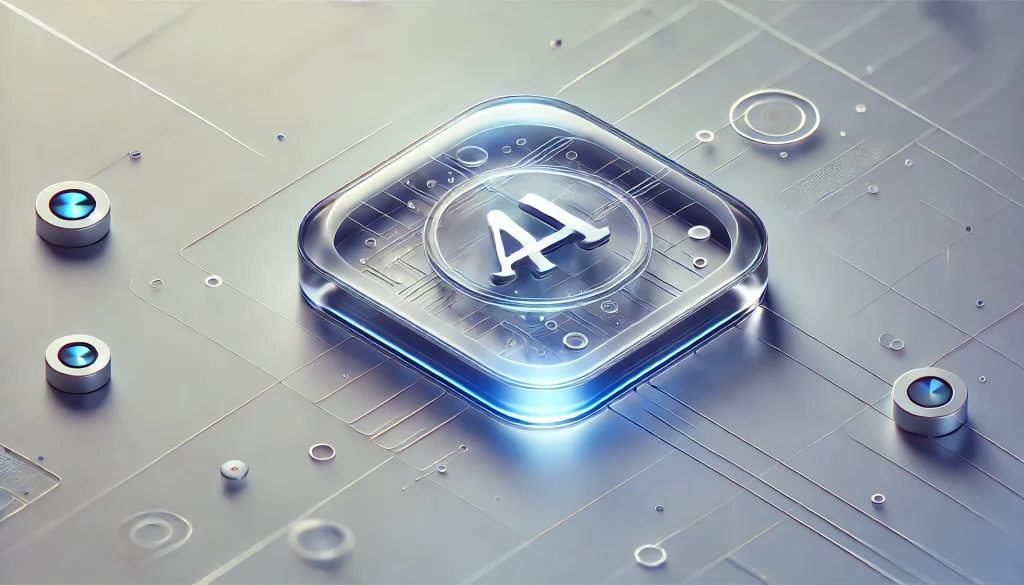
Making AI Explainable: Why Transparency Matters
Artificial Intelligence (AI) has become an integral part of our daily lives, revolutionizing industries and transforming the way we interact with technology. From virtual assistants on our smartphones to complex algorithms powering financial markets, AI is everywhere. But as these systems become more sophisticated and influential, a pressing question emerges: How can we ensure that AI remains transparent and accountable? This growing concern has given rise to the field of explainable AI (XAI), which aims to make AI systems more understandable to humans. In this blog post, we’ll delve into the importance of AI transparency and explore why it matters for individuals, businesses, and society as a whole.
The Black Box Dilemma
At the heart of the AI transparency issue lies the concept of the “black box.” Many AI systems, particularly deep learning models, operate in ways that are not easily interpretable by humans. These complex algorithms process vast amounts of data and make decisions based on patterns and relationships that may not be immediately apparent to us. While this approach can lead to impressive results, it also creates a significant problem: How can we trust decisions made by systems we don’t fully understand? This lack of transparency can have serious implications, especially in high-stakes scenarios like healthcare diagnostics, criminal justice, or autonomous vehicles. As AI continues to play a more prominent role in critical decision-making processes, the need for explainability becomes increasingly urgent.
The Ripple Effects of Opaque AI
The implications of non-transparent AI extend far beyond mere technical curiosity. When AI systems make decisions that affect people’s lives without clear explanations, it erodes public trust in technology and the institutions that deploy it. This erosion of trust can lead to widespread skepticism and resistance to AI adoption, potentially slowing down innovation and progress in fields where AI could bring significant benefits. Moreover, the lack of transparency makes it challenging to hold AI systems and their creators accountable for errors or biases. Without a clear understanding of how decisions are made, it becomes nearly impossible to identify and rectify problematic patterns or unintended consequences. This accountability gap raises serious ethical concerns, particularly when AI is used in sensitive areas like hiring, lending, or criminal sentencing.
The Push for Explainable AI
Recognizing these challenges, researchers and industry leaders are increasingly focusing on developing explainable AI systems. The goal of XAI is to create AI models that can not only make accurate predictions or decisions but also provide clear, understandable explanations for their outputs. This involves developing new algorithms and techniques that balance performance with interpretability. Some approaches include using simpler, more transparent models where possible, developing visualization tools to help humans understand complex AI decision processes, and creating AI systems that can generate natural language explanations for their actions. By making AI more explainable, we can foster greater trust, enable more effective human-AI collaboration, and ensure that AI systems align with human values and ethical standards.
The Benefits of Transparent AI
Embracing explainable AI offers numerous benefits across various sectors. In healthcare, transparent AI can help doctors understand and validate AI-assisted diagnoses, leading to more accurate and trustworthy medical decisions. In finance, explainable AI models can provide clear rationales for credit decisions, helping to prevent discrimination and ensure fair lending practices. For businesses, transparent AI can lead to more effective decision-making by allowing human experts to understand and validate AI recommendations. Moreover, explainable AI can accelerate innovation by making it easier for researchers and developers to identify and correct errors, improve model performance, and discover new insights. By demystifying AI, we can harness its full potential while maintaining human oversight and control.
Challenges in Achieving AI Transparency
While the benefits of explainable AI are clear, achieving true transparency in complex AI systems is no easy feat. One of the main challenges is the trade-off between model performance and interpretability. Often, the most accurate AI models are also the most complex and least explainable. Simplifying these models to make them more transparent can sometimes come at the cost of reduced accuracy or efficiency. Another challenge lies in translating technical explanations into terms that non-experts can understand. AI systems often operate on highly abstract mathematical principles that don’t easily map to human-understandable concepts. Researchers must find ways to bridge this gap without oversimplifying to the point of meaninglessness. Additionally, there’s the question of how much explanation is enough. Too little information may not provide sufficient insight, while too much could overwhelm users and obscure the most important factors.
The Role of Regulation in Promoting AI Transparency
As AI becomes more pervasive, governments and regulatory bodies are beginning to grapple with the need for oversight and standards in AI development and deployment. The European Union’s proposed AI Act, for example, includes provisions for transparency and explainability in high-risk AI applications. In the United States, various agencies are exploring guidelines for AI transparency in sectors like finance and healthcare. However, crafting effective regulations for AI transparency is a complex task. Policymakers must strike a delicate balance between promoting innovation and ensuring accountability. Overly stringent requirements could stifle AI development and put regions at a competitive disadvantage. On the other hand, insufficient regulation could leave the public vulnerable to the risks of opaque AI systems. Finding the right approach will require close collaboration between policymakers, industry experts, and researchers to develop standards that are both meaningful and practical.
The Human Factor: AI Literacy and Education
While technical solutions and regulations are crucial for achieving AI transparency, we must not overlook the importance of human understanding. As AI becomes more prevalent in our lives, there’s a growing need for widespread AI literacy. This involves educating the public about how AI works, its capabilities and limitations, and how to interpret AI-generated explanations. Schools and universities are beginning to incorporate AI education into their curricula, but we need broader efforts to reach adults as well. Companies deploying AI systems should invest in user education and provide clear, accessible information about how their AI works. Media outlets and public institutions also have a role to play in demystifying AI and promoting informed public discourse. By improving AI literacy, we can create a society that is better equipped to critically engage with AI systems, demand transparency where it matters, and make informed decisions about AI use.
The Future of Explainable AI
As research in explainable AI continues to advance, several promising trends are emerging. One exciting area is the development of “self-explaining” AI systems that can generate natural language explanations for their decisions in real-time. These systems aim to provide contextual, user-friendly explanations that adapt to the user’s level of expertise. Another trend is the integration of causal reasoning into AI models, which could help systems provide more intuitive explanations based on cause-and-effect relationships. Researchers are also exploring ways to combine multiple explanation techniques to provide more comprehensive and robust interpretability. As quantum computing matures, it may offer new avenues for creating more transparent AI systems that can handle complex computations while maintaining interpretability. While we’re still in the early stages of truly explainable AI, these developments offer hope for a future where AI transparency is the norm rather than the exception.
Case Studies: Transparency in Action
To better understand the impact of AI transparency, let’s look at some real-world examples where explainable AI has made a difference. In the financial sector, some banks are using explainable AI models for credit scoring that can provide clear reasons for loan approvals or denials. This transparency helps ensure fair lending practices and allows customers to understand how to improve their creditworthiness. In healthcare, researchers have developed explainable AI systems for cancer diagnosis that not only predict the presence of tumors but also highlight the specific image features that influenced the decision. This allows doctors to validate the AI’s findings and make more informed treatment decisions. In the automotive industry, explainable AI is being used in the development of self-driving cars to help engineers understand and improve the decision-making processes of autonomous vehicles. These examples demonstrate how AI transparency can enhance trust, improve outcomes, and accelerate innovation across various industries.
The Role of Open Source in Promoting AI Transparency
Open source initiatives play a crucial role in advancing AI transparency. By making AI algorithms and models publicly available, open source projects enable wider scrutiny and collaboration. This openness allows researchers, developers, and ethical hackers to examine AI systems for potential biases, security vulnerabilities, or unintended behaviors. Open source explainable AI tools and libraries, such as LIME (Local Interpretable Model-agnostic Explanations) and SHAP (SHapley Additive exPlanations), provide developers with accessible methods to add interpretability to their AI models. Moreover, open source fosters a culture of knowledge sharing and collaborative problem-solving, which is essential for tackling the complex challenges of AI transparency. As the field of explainable AI evolves, open source will likely continue to be a driving force in democratizing access to transparent AI technologies and promoting best practices in AI development.
Ethical Considerations in Explainable AI
As we strive for greater AI transparency, we must also grapple with the ethical implications of explainable AI. While transparency is generally seen as a positive goal, there are scenarios where full disclosure might not be desirable or even ethical. For instance, in healthcare applications, how do we balance the need for explainability with patient privacy concerns? In security and defense applications, how much transparency can we provide without compromising sensitive information or strategies? There’s also the question of how explanations might be manipulated or misused. Could bad actors use the insights gained from explainable AI to game the system or exploit vulnerabilities? Additionally, we must consider the potential psychological impacts of AI explanations on users. How do we provide explanations that are helpful and empowering rather than overwhelming or distressing? Navigating these ethical considerations will require ongoing dialogue between AI developers, ethicists, policymakers, and the public to establish guidelines and best practices for responsible AI transparency.
The Business Case for Transparent AI
For businesses, investing in explainable AI isn’t just about ethics or regulatory compliance—it’s also a smart business move. Transparent AI systems can provide a significant competitive advantage in several ways. First, they can enhance customer trust and loyalty. In an era where data privacy and algorithmic fairness are growing concerns, companies that can demonstrate the transparency and fairness of their AI systems are likely to win more customer confidence. Second, explainable AI can lead to better decision-making within organizations. When AI recommendations come with clear explanations, human decision-makers can more effectively combine AI insights with their own expertise and judgment. This can result in more accurate, efficient, and defensible decisions. Third, transparent AI systems are easier to debug, maintain, and improve. When developers can understand why an AI system is making certain decisions, they can more quickly identify and fix issues, leading to more robust and reliable AI applications. Finally, embracing AI transparency can help companies stay ahead of regulatory requirements and avoid potential legal issues related to opaque AI use. By investing in explainable AI now, businesses can future-proof their AI strategies and position themselves as responsible leaders in the AI revolution.
Conclusion
As we’ve explored throughout this blog post, the push for explainable AI is not just a technical challenge—it’s a societal imperative. The black box nature of many current AI systems poses significant risks to trust, accountability, and ethical use of AI technologies. By embracing transparency and investing in explainable AI, we can harness the full potential of artificial intelligence while maintaining human oversight and aligning AI systems with our values and ethical standards. The path to truly transparent AI is not an easy one. It requires ongoing research, collaboration between various stakeholders, thoughtful regulation, and a commitment to public education and AI literacy. However, the benefits of this journey are clear: more trustworthy AI systems, better decision-making, accelerated innovation, and a technology landscape that empowers rather than mystifies users. As we continue to integrate AI more deeply into our lives and societies, let’s prioritize transparency and work towards a future where AI’s decision-making processes are as clear and understandable as they are powerful and transformative.
Disclaimer: This blog post provides an overview of explainable AI based on current understanding and research. As the field of AI is rapidly evolving, some information may become outdated over time. While we strive for accuracy, we encourage readers to consult the latest research and expert opinions for the most up-to-date information. If you notice any inaccuracies in this post, please report them so we can correct them promptly.