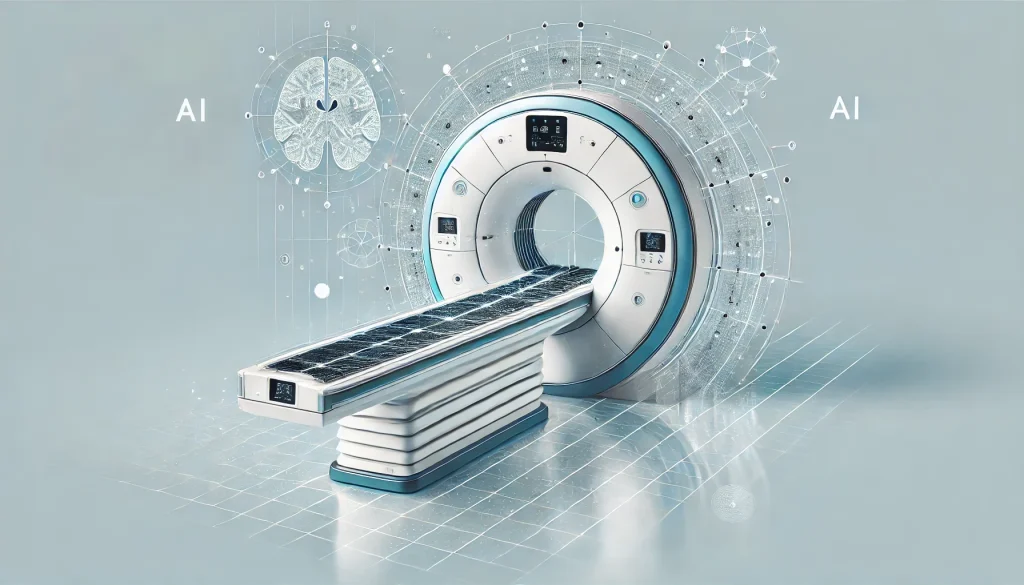
Medical Imaging: Using AI to See Inside the Body
Medical imaging has come a long way since the discovery of X-rays in 1895. From those early days of fuzzy black-and-white images, we’ve progressed to high-resolution 3D scans that can reveal the tiniest details of our internal organs. But the latest revolution in medical imaging isn’t just about sharper pictures – it’s about making those images smarter. Enter artificial intelligence (AI), the game-changing technology that’s transforming how we see inside the human body.
Imagine a world where a computer can spot a tumor that even the most experienced radiologist might miss. Or a future where AI can predict health issues before they become serious problems. This isn’t science fiction – it’s happening right now in hospitals and research labs around the globe. AI is enhancing every aspect of medical imaging, from how we capture images to how we interpret them. It’s making diagnoses faster, more accurate, and more accessible than ever before.
But what exactly is AI doing in medical imaging? How is it changing the way doctors work and patients receive care? And what does this mean for the future of healthcare? In this blog post, we’ll dive deep into the fascinating world of AI-powered medical imaging. We’ll explore the cutting-edge technologies that are revolutionizing the field, the challenges they’re helping to overcome, and the exciting possibilities they’re opening up for the future of medicine.
The Basics: What is AI in Medical Imaging?
Before we delve into the specifics, let’s start with a simple question: What do we mean when we talk about AI in medical imaging? At its core, AI in this context refers to computer systems that can perform tasks that typically require human intelligence. These tasks include visual perception, pattern recognition, and decision-making – all crucial elements in interpreting medical images.
Machine Learning and Deep Learning
Two key subfields of AI are particularly important in medical imaging: machine learning and deep learning. Machine learning algorithms can learn from and make predictions or decisions based on data. They improve their performance over time as they’re exposed to more information. Deep learning, a subset of machine learning, uses artificial neural networks inspired by the human brain to process data and create patterns for decision making.
In medical imaging, these AI technologies are applied in various ways. They can help enhance image quality, automatically detect and classify abnormalities, assist in treatment planning, and even predict patient outcomes. The goal is not to replace human experts, but to augment their capabilities, helping them work more efficiently and accurately.
How AI “Sees” Medical Images
When an AI system analyzes a medical image, it’s not “seeing” in the same way a human does. Instead, it’s processing the image as a complex set of data points. The AI has been trained on thousands or even millions of similar images, learning to recognize patterns and features associated with different conditions or anatomical structures.
For example, when analyzing a chest X-ray, an AI might break the image down into millions of pixels, each with its own brightness value. It then looks for patterns in these values that correspond to features it’s been trained to recognize – like the shape of a lung, the outline of the heart, or the telltale signs of pneumonia. This process happens incredibly quickly, often in just seconds, allowing for rapid analysis of large numbers of images.
The Impact of AI on Different Imaging Modalities
AI is making waves across all types of medical imaging, from X-rays to MRIs and beyond. Let’s take a closer look at how AI is enhancing some of the most common imaging modalities.
X-rays: The First Frontier
X-rays were the first form of medical imaging, and they’re still one of the most widely used. They’re quick, relatively inexpensive, and can provide valuable information about a wide range of conditions. However, interpreting X-rays can be challenging, especially when looking for subtle abnormalities.
This is where AI shines. AI algorithms can analyze X-rays in seconds, highlighting potential areas of concern for radiologists to review. They’re particularly good at detecting lung conditions like pneumonia or tuberculosis, as well as identifying fractures that might be easily overlooked. Some AI systems have even shown the ability to detect COVID-19 from chest X-rays, potentially speeding up diagnosis in emergency situations.
CT Scans: Slicing Through Complexity
Computed Tomography (CT) scans provide detailed 3D images of the body, but they also generate huge amounts of data. A single CT scan can produce hundreds of individual images, which can take a radiologist significant time to review thoroughly.
AI can help by quickly analyzing these images and flagging potential abnormalities. It can assist in detecting and measuring tumors, identifying signs of stroke or internal bleeding, and even helping to plan radiation therapy treatments. Some AI systems can also reduce the amount of radiation needed for a CT scan, making the procedure safer for patients.
MRI: Magnetic Resonance meets Artificial Intelligence
Magnetic Resonance Imaging (MRI) provides exquisite detail of soft tissues in the body, but it can be a time-consuming and expensive procedure. AI is helping to address these challenges in several ways.
First, AI can help optimize MRI protocols, potentially reducing scan times without sacrificing image quality. This not only improves patient comfort but also increases the number of scans that can be performed in a day. AI can also assist in image reconstruction, potentially allowing for shorter scan times or higher resolution images.
In image analysis, AI is proving particularly useful in neurological applications. AI algorithms can help detect and classify brain tumors, identify early signs of neurodegenerative diseases like Alzheimer’s, and even predict which patients with mild cognitive impairment are likely to progress to Alzheimer’s disease.
Ultrasound: Real-Time AI Assistance
Ultrasound imaging is unique in that it provides real-time images, making it invaluable for guiding procedures and monitoring developing fetuses. However, the quality of ultrasound images can vary significantly depending on the skill of the operator and the patient’s body composition.
AI is helping to level the playing field by assisting in image acquisition and interpretation. Some AI systems can guide less experienced operators to obtain the best possible images. Others can automatically measure fetal biometrics or detect potential abnormalities in real-time.
In cardiac ultrasound, AI is proving particularly useful in automatically calculating measures of heart function, potentially allowing for earlier detection of heart disease.
AI in Cancer Detection and Diagnosis
One of the most promising applications of AI in medical imaging is in the field of cancer detection and diagnosis. Early detection is crucial in improving cancer outcomes, and AI is proving to be a powerful tool in this area.
Breast Cancer Screening
Mammography is the primary screening tool for breast cancer, but interpreting mammograms can be challenging. Dense breast tissue can obscure potential tumors, and the sheer volume of screenings can lead to fatigue among radiologists.
AI algorithms have shown impressive results in detecting breast cancer on mammograms. Some studies have found that AI can perform as well as or better than human radiologists in identifying malignant tumors. AI can also help classify breast density, which is an important risk factor for breast cancer.
Perhaps most excitingly, some AI systems have shown the ability to predict a woman’s risk of developing breast cancer in the future based on her mammograms. This could allow for more personalized screening schedules and earlier interventions for high-risk patients.
Lung Cancer Detection
Lung cancer is often detected late, making it one of the deadliest forms of cancer. AI is showing promise in improving early detection through analysis of chest CT scans.
AI algorithms can detect small lung nodules that might be easily missed by human observers. They can also help classify these nodules as benign or potentially malignant, reducing unnecessary follow-up procedures for benign nodules while ensuring closer monitoring of suspicious ones.
Some AI systems are even able to predict a person’s risk of developing lung cancer based on their CT scans, potentially allowing for earlier and more targeted screening of high-risk individuals.
Prostate Cancer Diagnosis
Prostate cancer diagnosis typically involves a combination of blood tests, physical exams, and imaging studies. AI is helping to improve the accuracy of prostate cancer diagnosis and staging through analysis of MRI images.
AI algorithms can help identify suspicious areas on prostate MRIs that warrant further investigation. They can also assist in determining the aggressiveness of prostate tumors, potentially reducing the need for invasive biopsies and helping to guide treatment decisions.
Beyond Diagnosis: AI in Treatment Planning and Monitoring
While much of the focus on AI in medical imaging has been on diagnosis, its potential extends far beyond that. AI is also making significant impacts in treatment planning and monitoring.
Radiation Therapy Planning
Radiation therapy is a common treatment for many types of cancer, but planning the treatment can be a complex and time-consuming process. AI is helping to streamline this process in several ways.
AI algorithms can automatically segment organs and tumors on CT or MRI scans, a task that traditionally required manual contouring by radiation oncologists. This not only saves time but can also improve consistency between different treatment planners.
Some AI systems can even generate entire radiation treatment plans, optimizing the radiation dose to maximize tumor coverage while minimizing damage to healthy tissues. While these plans still require review and approval by human experts, they can significantly speed up the planning process.
Surgical Planning
In the realm of surgery, AI-enhanced imaging is helping surgeons plan and execute complex procedures with greater precision. By analyzing pre-operative scans, AI can help create detailed 3D models of a patient’s anatomy, allowing surgeons to virtually plan their approach before making a single incision.
In neurosurgery, for example, AI can help map out the safest route to a brain tumor, taking into account critical structures that need to be avoided. In orthopedic surgery, AI can assist in planning joint replacements, helping to ensure optimal implant positioning.
Treatment Response Monitoring
Once treatment has begun, AI can play a crucial role in monitoring its effectiveness. By analyzing follow-up scans, AI algorithms can detect subtle changes that might indicate whether a treatment is working or not.
In cancer treatment, for instance, AI can help measure changes in tumor size and composition over time. This can allow for earlier detection of treatment resistance, potentially allowing doctors to switch to alternative therapies before the cancer has a chance to progress further.
The Challenges and Limitations of AI in Medical Imaging
While the potential of AI in medical imaging is enormous, it’s important to acknowledge that there are still significant challenges and limitations to overcome.
Data Quality and Quantity
AI algorithms are only as good as the data they’re trained on. In medical imaging, this presents several challenges. First, there’s the issue of data quantity. Training effective AI models often requires large datasets, which can be difficult to obtain in healthcare due to privacy concerns and the rarity of certain conditions.
Then there’s the question of data quality. Medical images can vary significantly depending on the equipment used, the imaging protocol followed, and even the individual patient’s anatomy. Ensuring that AI models can generalize well across different settings and populations is a major challenge.
Interpretability and Trust
Many AI models, particularly deep learning models, operate as “black boxes.” They can make highly accurate predictions, but it’s often not clear how they arrived at those predictions. This lack of interpretability can make it difficult for healthcare providers to trust and act on AI-generated insights.
Efforts are underway to develop more interpretable AI models, as well as to create tools that can explain AI decisions in ways that are meaningful to healthcare providers. However, this remains an active area of research and development.
Regulatory and Ethical Considerations
As with any new medical technology, AI in medical imaging must navigate a complex regulatory landscape. Ensuring the safety and efficacy of AI-powered medical devices is crucial, but traditional approval processes may not be well-suited to AI systems that continually learn and evolve.
There are also important ethical considerations to address. How do we ensure that AI systems are fair and unbiased across different patient populations? How do we protect patient privacy when AI systems require large amounts of data to function effectively? These are questions that the medical community, policymakers, and society at large will need to grapple with as AI becomes more prevalent in healthcare.
The Future of AI in Medical Imaging
Despite these challenges, the future of AI in medical imaging looks incredibly bright. As technology continues to advance, we can expect to see even more innovative applications of AI in this field.
Multimodal AI
One exciting area of development is multimodal AI, which can integrate information from multiple imaging modalities as well as other sources of patient data. For example, an AI system might combine information from a patient’s CT scan, blood tests, and electronic health record to provide a more comprehensive assessment of their health status.
Personalized Medicine
AI could play a crucial role in advancing personalized medicine. By analyzing large datasets of medical images and patient outcomes, AI could help predict which treatments are likely to be most effective for individual patients based on their unique characteristics.
Automated Reporting
While AI is already assisting in image analysis, future systems may be able to generate entire radiology reports automatically. This could free up radiologists to focus on more complex cases and direct patient care.
Predictive Imaging
Perhaps most excitingly, AI may eventually allow us to predict future health issues before they occur. By analyzing patterns in medical images over time, AI could potentially identify early warning signs of diseases long before they would be detectable by current methods.
Conclusion
As we’ve explored in this blog post, AI is ushering in a new era in medical imaging. From improving image quality to enhancing diagnosis and treatment planning, AI is revolutionizing how we see inside the human body.
While there are certainly challenges to overcome, the potential benefits of AI in medical imaging are enormous. Faster, more accurate diagnoses. More personalized treatment plans. Earlier detection of diseases. These advances could save countless lives and improve the quality of care for millions of patients around the world.
As we look to the future, it’s clear that AI will play an increasingly important role in healthcare. But it’s important to remember that AI is a tool, not a replacement for human expertise. The most effective healthcare systems of the future will likely be those that successfully integrate AI technologies with the knowledge, experience, and empathy of human healthcare providers.
The journey of AI in medical imaging is just beginning, and the most exciting developments may still be ahead. As researchers, clinicians, and technologists continue to push the boundaries of what’s possible, we can look forward to a future where seeing inside the human body is not just clearer, but smarter than ever before.
Disclaimer: This blog post is intended for informational purposes only and should not be considered medical advice. While we strive for accuracy, medical knowledge is constantly evolving, and individual medical situations can vary greatly. Always consult with qualified healthcare professionals for medical advice and treatment. If you notice any inaccuracies in this post, please report them so we can correct them promptly.