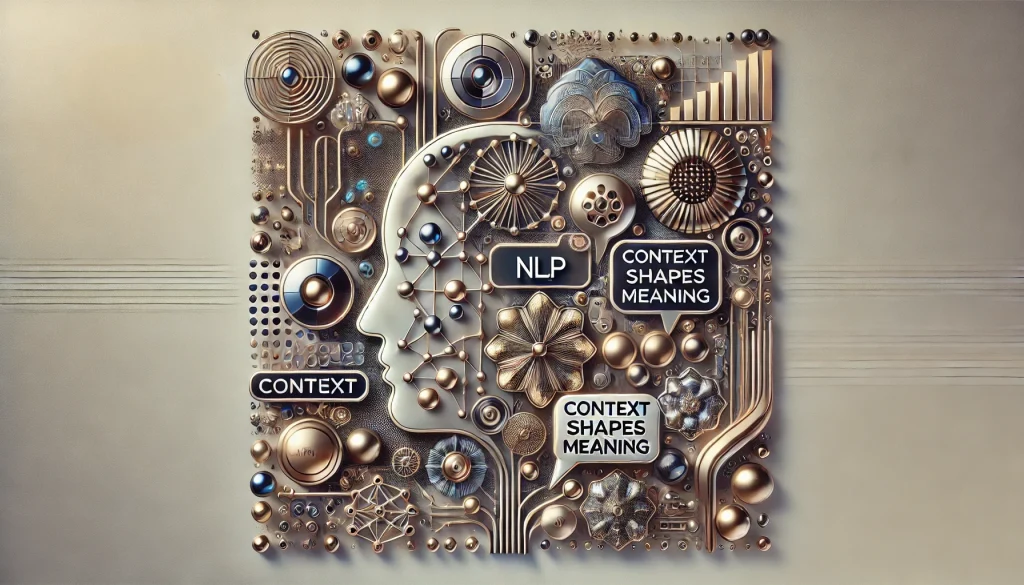
Pragmatics in NLP: How Context Shapes Language Meaning
Have you ever wondered why your smart speaker sometimes misunderstands you, or why machine translation can produce hilarious (and sometimes embarrassing) results? The answer lies in a fascinating field called pragmatics, which plays a crucial role in Natural Language Processing (NLP). Today, we’re diving deep into the world of pragmatics and exploring how context shapes the meaning of language in NLP. Buckle up, language enthusiasts and tech aficionados – we’re in for an exciting ride!
What is Pragmatics, Anyway?
Let’s start with the basics. Pragmatics is the study of how context contributes to meaning. It’s all about understanding language beyond just the literal definitions of words. Think of it as the “reading between the lines” of linguistics.
Why does pragmatics matter in everyday communication?
Imagine you’re at a dinner party, and someone says, “Can you pass the salt?” Now, technically, they’re asking if you’re capable of passing the salt. But in reality, they’re politely requesting that you actually do it. That’s pragmatics in action! It’s the difference between what’s said and what’s meant.
In human communication, we use pragmatics all the time without even realizing it. We interpret tone, consider the speaker’s intentions, and factor in social context. It’s what allows us to understand sarcasm, pick up on subtle hints, and navigate the complex world of human interaction.
The Pragmatics Challenge in NLP
Now, let’s shift gears and talk about why pragmatics is such a big deal in Natural Language Processing. NLP is all about enabling machines to understand, interpret, and generate human language. Sounds simple enough, right? Well, not quite.
The literal meaning trap
Here’s the thing: computers are excellent at processing literal meanings. They can quickly look up definitions, analyze grammar, and even identify parts of speech. But when it comes to understanding context and implied meanings – the realm of pragmatics – things get tricky.
Let’s consider an example. Imagine you tell your AI assistant, “It’s raining cats and dogs out there!” A system without pragmatic understanding might start searching for felines and canines falling from the sky. Amusing, but not very helpful!
This is why incorporating pragmatics into NLP is so crucial. It’s the key to making machines truly understand language the way humans do.
Context is King: The Role of Pragmatics in NLP
So, how exactly does pragmatics help shape language meaning in NLP? Let’s break it down.
Disambiguating language
One of the biggest challenges in NLP is dealing with ambiguity. Words and phrases can have multiple meanings, and without context, it’s impossible to know which one is intended. This is where pragmatics comes to the rescue.
For example, consider the word “bank.” It could refer to a financial institution, the side of a river, or even the act of tilting an aircraft. By analyzing the context – the surrounding words, the topic of conversation, or even the user’s location – an NLP system with pragmatic understanding can determine the correct meaning.
Understanding intent
Pragmatics also helps NLP systems grasp the underlying intent behind a user’s words. This is particularly important in applications like chatbots and virtual assistants.
For instance, if a user says, “I’m feeling under the weather,” a pragmatically-aware system would understand that this is likely a complaint about being ill, rather than a literal statement about their position relative to meteorological conditions. It might then offer appropriate responses, such as suggesting rest or inquiring if the user needs medical advice.
The Challenges of Implementing Pragmatics in NLP
While the benefits of incorporating pragmatics into NLP are clear, it’s not without its challenges. Let’s explore some of the hurdles that researchers and developers face.
Cultural context
One of the biggest challenges is accounting for cultural differences in language use. Pragmatic norms can vary widely between cultures, making it difficult to create a one-size-fits-all solution.
For example, in some cultures, it’s common to refuse an offer several times before accepting, even if you actually want to accept right away. An NLP system designed for one cultural context might misinterpret this behavior if applied in another.
Handling non-literal language
Another significant challenge is dealing with figurative language, such as idioms, metaphors, and sarcasm. These expressions often rely heavily on shared cultural knowledge and context, making them particularly tricky for machines to interpret correctly.
Take the phrase “break a leg.” To a system without pragmatic understanding, this might be interpreted as a threat or a suggestion to cause harm. But of course, we know it’s actually used to wish someone good luck, particularly in theatrical contexts.
Pragmatics in Action: Real-World NLP Applications
Now that we’ve covered the basics and challenges, let’s look at some exciting ways pragmatics is being applied in NLP today.
Sentiment analysis
Sentiment analysis is all about determining the emotional tone behind a piece of text. It’s widely used in social media monitoring, customer service, and market research. By incorporating pragmatic understanding, these systems can pick up on subtle cues and context that might indicate sarcasm, irony, or understated emotions.
For instance, the statement “Great, just what I needed today” could be genuinely positive or deeply sarcastic, depending on the context. A pragmatically-aware system would be better equipped to make this distinction.
Machine translation
Machine translation has come a long way, but it still often stumbles when it comes to idiomatic expressions or culturally-specific references. By integrating pragmatic understanding, these systems can produce more natural and contextually appropriate translations.
Consider the French expression “Il pleut comme vache qui pisse” (literally, “It’s raining like a peeing cow”). A pragmatically-aware translation system might render this as “It’s raining cats and dogs” in English, capturing the intended meaning rather than producing a literal but nonsensical translation.
The Future of Pragmatics in NLP
As we look to the future, the role of pragmatics in NLP is only set to grow. Here are some exciting developments on the horizon.
Multimodal NLP
Future NLP systems are likely to incorporate multiple modes of communication, including text, speech, and even visual cues. This multimodal approach will provide richer context, allowing for more nuanced pragmatic understanding.
Imagine a virtual assistant that can not only understand your words but also interpret your tone of voice and facial expressions. This could lead to much more natural and context-aware interactions.
Personalized pragmatics
Another promising area is the development of personalized pragmatic models. These systems would learn individual users’ communication styles, preferences, and contexts over time, allowing for increasingly accurate interpretation of their language use.
For example, a system might learn that when you say “I’m fine,” you actually mean you’re upset and need support. This level of personalized understanding could revolutionize areas like mental health support and personal assistant technologies.
Ethical Considerations in Pragmatic NLP
As we push the boundaries of what’s possible with pragmatic NLP, it’s crucial that we also consider the ethical implications of these advancements.
Privacy concerns
The more context an NLP system has, the better it can understand pragmatic nuances. However, this also raises important questions about data privacy. How much information about a user’s personal context should these systems be allowed to access and store?
Bias and fairness
Like all AI systems, NLP models can inadvertently perpetuate biases present in their training data. When it comes to pragmatics, there’s a risk of reinforcing cultural stereotypes or misinterpreting communication styles that differ from the dominant norms in the training data.
Ensuring fairness and inclusivity in pragmatic NLP systems will be an ongoing challenge that requires diverse perspectives and careful consideration.
Conclusion
As we’ve explored in this deep dive, pragmatics plays a crucial role in shaping language meaning in NLP. It’s the key to bridging the gap between literal, machine-like language processing and the rich, context-dependent way humans communicate.
While there are certainly challenges to overcome, the potential benefits of incorporating pragmatic understanding into NLP systems are enormous. From more natural human-computer interactions to more accurate sentiment analysis and machine translation, pragmatics has the power to revolutionize the way we interact with language technology.
As we move forward, it’s exciting to imagine a future where our devices truly understand not just what we say, but what we mean. The path to get there may be complex, but it’s undoubtedly a journey worth taking.
So, the next time you find yourself frustrated with a virtual assistant or chuckling at a comically literal translation, remember: there’s a whole world of pragmatics working behind the scenes to make these interactions smoother and more natural. And who knows? Maybe one day, your AI assistant will even be able to appreciate your witty sarcasm!
Disclaimer: This article aims to provide an overview of pragmatics in NLP based on current understanding and research. As this is a rapidly evolving field, some information may become outdated over time. We encourage readers to consult recent academic publications and industry reports for the most up-to-date information. If you notice any inaccuracies, please report them so we can correct them promptly.