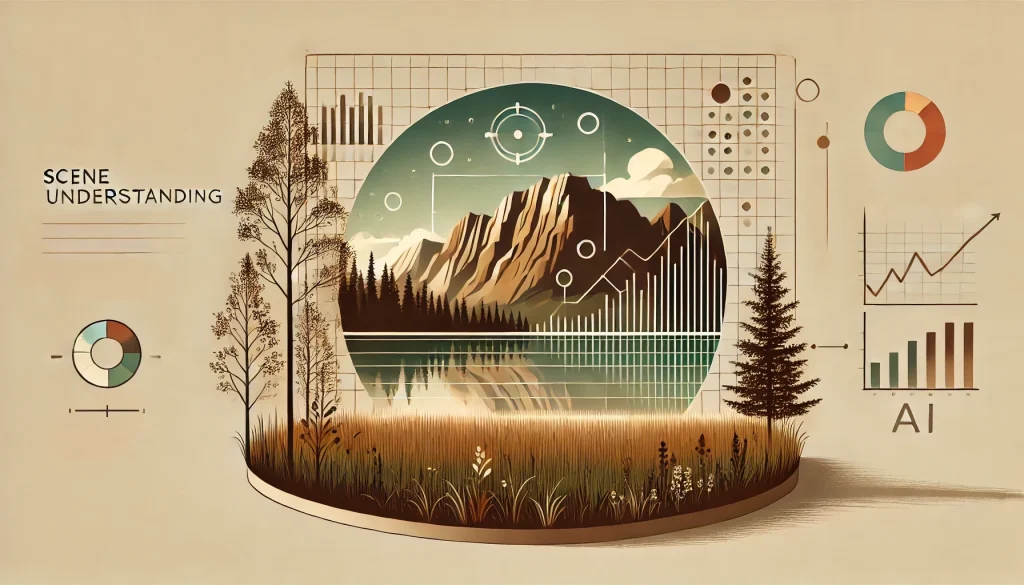
Scene Understanding: AI That Knows What’s Happening in Images
Imagine you’re at a concert, your favorite band is on stage, and the crowd is going wild. You snap a photo and upload it to your social media. Instantly, your phone recognizes the band, identifies the concert venue, and even tags your friends in the crowd. Welcome to the future of AI and scene understanding! This incredible technology is revolutionizing the way we interact with images, making our digital experiences richer, smarter, and more intuitive. But how exactly does it work? Let’s dive deep into the fascinating world of scene understanding in artificial intelligence.
What is Scene Understanding?
Definition and Basics
Scene understanding is a subfield of computer vision that involves interpreting and making sense of what’s happening in a scene depicted in an image or a video. This includes recognizing objects, understanding their relationships, and even predicting what might happen next. Essentially, it’s about teaching machines to see and comprehend the world as humans do.
Why It Matters
Why is scene understanding so important? Think about the endless applications: autonomous driving, where cars need to understand their surroundings to navigate safely; healthcare, where AI can analyze medical images to assist in diagnoses; and social media, where automatic tagging and content moderation enhance user experiences. Scene understanding makes these and many other technologies possible, driving innovation and improving lives.
How Does Scene Understanding Work?
Machine Learning and Deep Learning
At the core of scene understanding is machine learning, particularly deep learning. These techniques involve training neural networks on vast datasets of images so they can learn to recognize patterns and features. Convolutional Neural Networks (CNNs) are especially crucial here, as they are designed to process and understand image data.
Data Collection and Annotation
For AI to understand scenes accurately, it needs a lot of data. This data comes from various sources, including public image repositories, user-generated content, and specialized datasets created by researchers. However, the data alone isn’t enough. It must be meticulously annotated, meaning each element in the images is labeled with what it is and its relevance in the scene. This labor-intensive process is critical for training effective models.
Feature Extraction and Classification
Once the data is ready, the AI begins to learn by extracting features from the images. Features could be anything from edges and textures to colors and shapes. The neural network learns to identify these features and classify objects within the scene. For instance, in a street scene, the AI would recognize cars, pedestrians, traffic lights, and buildings, each with its own set of characteristics.
The Role of Context in Scene Understanding
Contextual Analysis
Understanding individual objects isn’t enough; context is crucial. For example, recognizing a stop sign is one thing, but understanding that it means vehicles should stop is another. Contextual analysis allows AI to interpret scenes more holistically, considering the relationships between objects and their roles within the scene.
Semantic Segmentation
Semantic segmentation is a technique used to partition an image into regions that correspond to different objects or parts of objects. This helps AI to understand not just what is in the image, but also where it is located and how it interacts with other elements. For example, in a beach scene, the AI can segment the sky, ocean, sand, and people, providing a detailed understanding of the environment.
Real-World Applications
Autonomous Vehicles
One of the most exciting applications of scene understanding is in autonomous vehicles. Self-driving cars rely heavily on their ability to interpret their surroundings accurately. They use a combination of cameras, LiDAR, and other sensors to perceive the environment, recognize objects like other vehicles, pedestrians, and obstacles, and make real-time decisions. This technology promises to revolutionize transportation, making it safer and more efficient.
Healthcare
In healthcare, scene understanding is making significant strides. AI systems can analyze medical images, such as X-rays, MRIs, and CT scans, to detect anomalies and assist in diagnoses. For example, AI can help identify tumors, fractures, and other conditions with remarkable accuracy, providing valuable support to medical professionals and potentially saving lives.
Retail and E-commerce
In the retail and e-commerce sectors, scene understanding is enhancing customer experiences. AI can analyze product images to recommend similar items, enable visual search, and even create virtual fitting rooms where customers can see how clothes would look on them. This technology is transforming how we shop online, making it more interactive and personalized.
Security and Surveillance
Security and surveillance systems are also benefiting from scene understanding. AI can analyze video feeds to detect suspicious activities, identify individuals, and monitor crowds. This enhances security in public places, helps in crime prevention, and provides valuable data for law enforcement agencies.
Challenges in Scene Understanding
Complexity and Variability
One of the biggest challenges in scene understanding is the sheer complexity and variability of real-world scenes. Scenes can be cluttered, objects can be occluded, and lighting conditions can vary widely. Teaching AI to handle these variations and still accurately interpret scenes is a formidable task.
Data Privacy and Ethical Concerns
The use of vast amounts of image data raises significant privacy and ethical concerns. Collecting and storing personal images, especially without consent, can lead to privacy violations. It’s essential to develop and adhere to ethical guidelines and regulations to ensure that scene understanding technologies are used responsibly and respect individuals’ privacy.
Computational Requirements
Training and deploying scene understanding models require substantial computational resources. High-performance GPUs, large memory capacities, and efficient algorithms are necessary to process the massive amounts of data involved. This can be a barrier for smaller organizations and researchers with limited resources.
Future of Scene Understanding
Advancements in AI
The future of scene understanding looks promising, with continuous advancements in AI and machine learning. Researchers are developing more sophisticated models that can handle greater complexity and variability. Transfer learning, where models trained on one task can be adapted for another, is also making significant progress, reducing the need for vast amounts of labeled data.
Integration with Other Technologies
Scene understanding is expected to integrate more seamlessly with other technologies, such as augmented reality (AR) and virtual reality (VR). Imagine AR applications that can understand and interact with the real world in real-time, or VR experiences that provide highly realistic and immersive environments based on accurate scene interpretation.
Broadening Accessibility
Efforts are underway to make scene understanding technology more accessible. Open-source frameworks and tools are being developed, allowing a wider range of researchers and developers to experiment with and contribute to this field. This democratization of technology will likely accelerate innovation and lead to new and exciting applications.
Conclusion
Scene understanding is a groundbreaking field in AI that’s transforming how machines perceive and interact with the world. From self-driving cars to healthcare, retail, and security, its applications are vast and varied. While challenges remain, the future holds immense potential for further advancements and integration with other emerging technologies. As we continue to develop and refine these systems, we can look forward to a world where AI not only sees but truly understands the scenes around us, making our lives smarter, safer, and more connected.
Disclaimer: The information provided in this blog is for educational purposes only and may not reflect the latest advancements in AI technology. Please report any inaccuracies so we can correct them promptly.