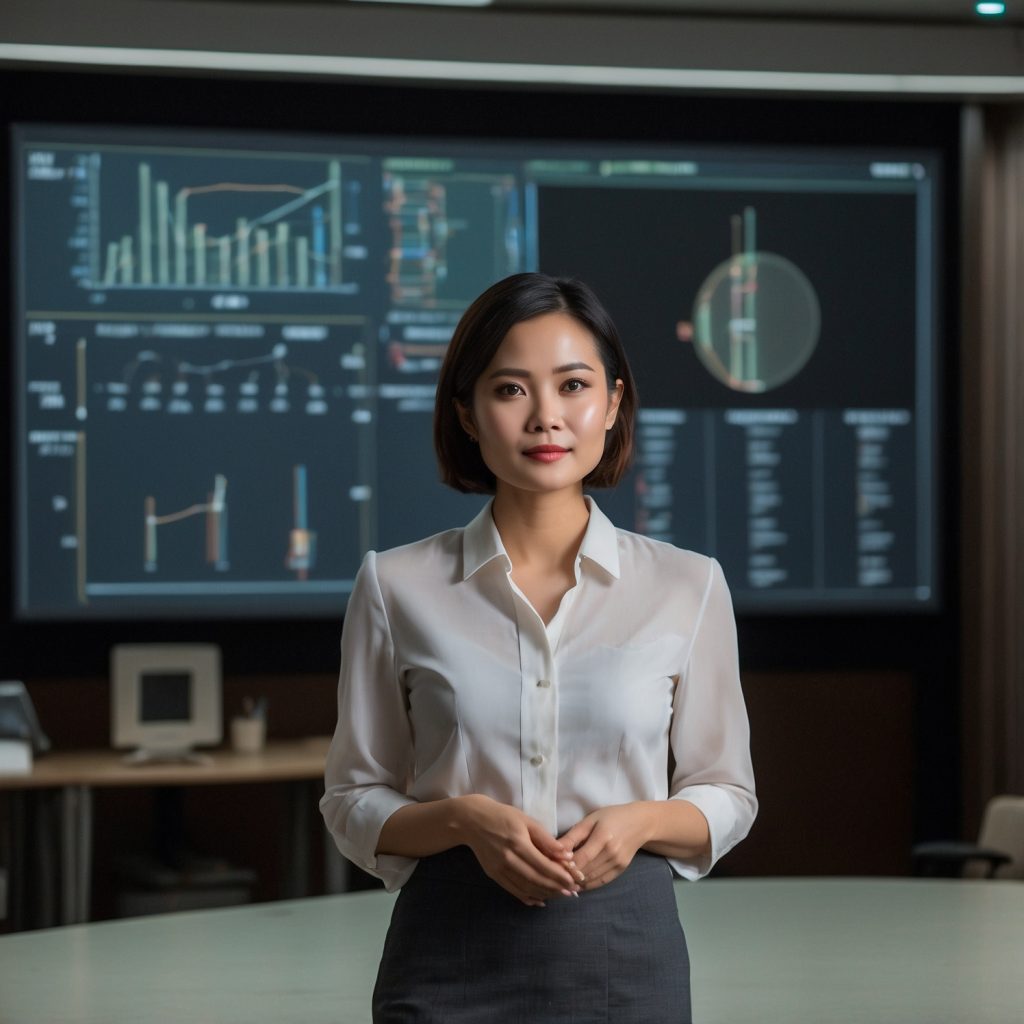
The Role of AI in Predictive Analytics
Predictive analytics has revolutionized the way businesses operate, offering unprecedented insights into future trends, behaviors, and outcomes. At the heart of this transformation lies Artificial Intelligence (AI), a technology that has taken predictive analytics to new heights. In this blog, we will explore how AI enhances predictive analytics, the benefits it brings, and its applications across various industries. Let’s delve into the fascinating world of AI-driven predictive analytics and understand its pivotal role in shaping the future.
Understanding Predictive Analytics
What is Predictive Analytics?
Predictive analytics involves using historical data, statistical algorithms, and machine learning techniques to predict future outcomes. It’s about identifying patterns and trends that can inform decision-making. By analyzing past data, businesses can forecast future events, reduce risks, and seize opportunities. This approach is not limited to any specific field; it is widely used in marketing, finance, healthcare, and beyond.
The Importance of Historical Data
Historical data serves as the foundation for predictive analytics. It provides the raw material from which patterns and trends are extracted. The more extensive and accurate the historical data, the better the predictions. This data includes various forms of information such as sales records, customer interactions, market trends, and more. By leveraging this data, predictive analytics can generate actionable insights that drive strategic decisions.
The Intersection of AI and Predictive Analytics
How AI Enhances Predictive Analytics
AI brings several advantages to predictive analytics, making it more efficient and accurate. Machine learning, a subset of AI, enables predictive models to learn from data and improve over time. This self-learning capability allows AI systems to handle vast amounts of data, identify complex patterns, and make more accurate predictions than traditional statistical methods. AI’s ability to process and analyze data at high speed significantly enhances the predictive analytics process.
AI Algorithms in Predictive Analytics
AI utilizes various algorithms to enhance predictive analytics, including regression models, decision trees, neural networks, and clustering techniques. These algorithms can analyze data from multiple sources, identify correlations, and make predictions with high accuracy. For instance, neural networks are particularly effective in recognizing patterns in large datasets, making them invaluable for predictive tasks in fields like finance and healthcare.
Benefits of AI-Driven Predictive Analytics
Improved Accuracy
One of the most significant benefits of AI in predictive analytics is improved accuracy. Traditional methods often struggle with the complexity and volume of modern data. AI, with its advanced algorithms, can handle large datasets and uncover intricate patterns, leading to more accurate predictions. This accuracy is crucial for businesses making strategic decisions based on these predictions.
Faster Data Processing
AI-driven predictive analytics can process data much faster than traditional methods. This speed allows businesses to analyze data in real-time and make timely decisions. For example, in the stock market, where conditions change rapidly, the ability to process and analyze data quickly can provide a competitive edge.
Enhanced Decision-Making
By providing accurate and timely insights, AI-driven predictive analytics enhances decision-making. Businesses can make informed decisions based on data-driven insights rather than relying on intuition or guesswork. This leads to better outcomes, such as increased sales, improved customer satisfaction, and reduced operational costs.
Scalability
AI systems are highly scalable, making them suitable for businesses of all sizes. Whether a small startup or a large corporation, AI-driven predictive analytics can be tailored to meet specific needs. This scalability ensures that businesses can grow and adapt their predictive analytics capabilities as they expand.
Applications of AI in Predictive Analytics
Marketing
In marketing, AI-driven predictive analytics is used to understand customer behavior, predict trends, and personalize marketing campaigns. By analyzing customer data, businesses can identify which products or services are likely to be in demand and tailor their marketing strategies accordingly. This personalized approach enhances customer engagement and increases conversion rates.
Finance
In the finance sector, predictive analytics is used for risk assessment, fraud detection, and investment forecasting. AI can analyze vast amounts of financial data to identify potential risks and opportunities. For example, banks use predictive analytics to assess the creditworthiness of loan applicants, while investment firms use it to predict stock market trends.
Healthcare
AI-driven predictive analytics is transforming healthcare by enabling early diagnosis, personalized treatment plans, and efficient resource allocation. By analyzing patient data, AI can predict the likelihood of diseases and recommend preventive measures. This proactive approach improves patient outcomes and reduces healthcare costs.
Supply Chain Management
In supply chain management, predictive analytics helps businesses forecast demand, optimize inventory levels, and improve logistics. AI can analyze data from various sources, such as sales records and market trends, to predict future demand. This allows businesses to manage their inventory more efficiently and reduce costs associated with overstocking or stockouts.
Customer Service
AI-driven predictive analytics is enhancing customer service by enabling businesses to anticipate customer needs and respond proactively. By analyzing customer interactions, businesses can predict common issues and provide timely solutions. This improves customer satisfaction and loyalty, leading to increased retention rates.
Challenges and Future Trends
Data Quality and Privacy
One of the primary challenges in AI-driven predictive analytics is ensuring data quality and privacy. Inaccurate or incomplete data can lead to incorrect predictions, while privacy concerns can limit the availability of data. Businesses must invest in data quality management and adhere to privacy regulations to overcome these challenges.
Integration with Existing Systems
Integrating AI-driven predictive analytics with existing systems can be complex and costly. Businesses need to ensure that their IT infrastructure can support advanced AI algorithms and handle large datasets. This may require significant investments in technology and training.
Future Trends
The future of AI-driven predictive analytics looks promising, with several trends on the horizon. Advances in AI algorithms and computing power will further enhance predictive accuracy and speed. The integration of AI with other technologies, such as the Internet of Things (IoT) and blockchain, will open up new possibilities for predictive analytics. Additionally, the increasing availability of big data will provide more opportunities for businesses to leverage predictive analytics for strategic decision-making.
The role of AI in predictive analytics is transformative, offering businesses unparalleled insights into future trends and behaviors. By enhancing accuracy, speed, and decision-making, AI-driven predictive analytics is helping businesses across various industries to stay ahead of the competition. Despite challenges related to data quality, privacy, and integration, the future of AI in predictive analytics looks bright, with continuous advancements and new applications emerging. As businesses continue to embrace AI-driven predictive analytics, they can expect to unlock new opportunities, drive innovation, and achieve sustainable growth.