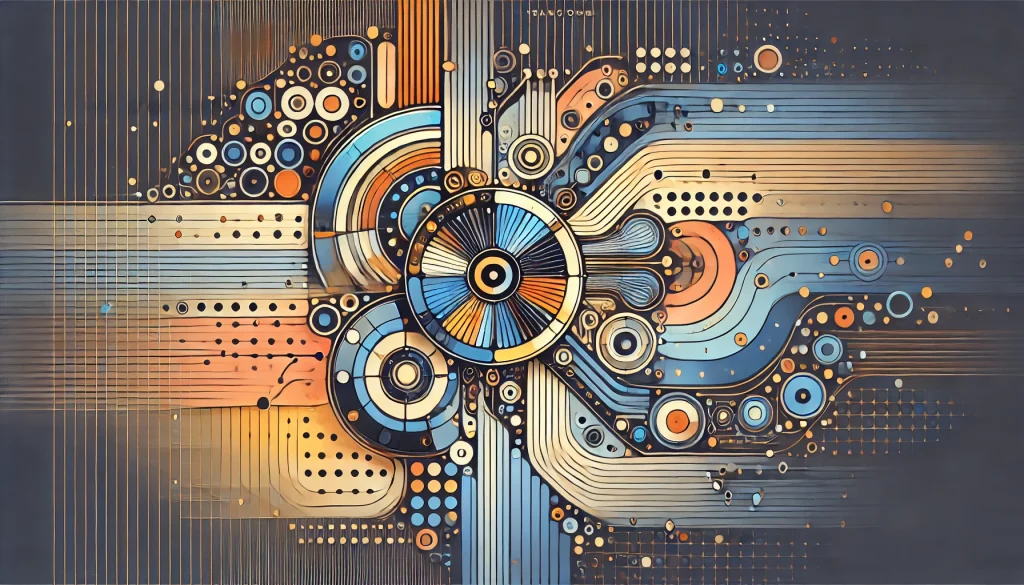
Transformers: The Future of NLP?
Natural Language Processing (NLP) has experienced remarkable advancements over the past few years, and at the heart of this evolution lies the Transformer architecture. As we delve into the intricacies of Transformers and their impact on NLP, we’ll uncover how this revolutionary technology is shaping the future of language understanding and generation.
Understanding Transformers
To grasp the significance of Transformers, we first need to understand what they are and how they work. Introduced by Vaswani et al. in 2017, Transformers represent a paradigm shift in NLP. Unlike previous models that relied heavily on recurrent neural networks (RNNs) or convolutional neural networks (CNNs), Transformers use a mechanism known as self-attention. This allows them to process entire sequences of data simultaneously rather than sequentially, which significantly speeds up computation and improves the handling of long-range dependencies in text.
Transformers consist of an encoder-decoder architecture. The encoder processes the input text, while the decoder generates the output text. Both components are made up of layers of self-attention and feed-forward neural networks. Self-attention enables the model to weigh the importance of different words in a sentence when making predictions, allowing for a more nuanced understanding of context.
The Rise of BERT and GPT
Two of the most influential models built on the Transformer architecture are BERT (Bidirectional Encoder Representations from Transformers) and GPT (Generative Pre-trained Transformer). These models have set new benchmarks in NLP tasks, demonstrating the power and versatility of Transformers.
BERT was introduced by Google in 2018 and quickly became a cornerstone in the field. It employs a bidirectional approach, meaning it reads text both left-to-right and right-to-left, capturing context from all directions. This enables BERT to understand the meaning of words based on their surrounding context more effectively than previous models. BERT excels in tasks like question answering, sentiment analysis, and named entity recognition.
GPT, on the other hand, was developed by OpenAI and focuses on generative tasks. The latest iteration, GPT-4, has showcased impressive abilities in text generation, translation, summarization, and more. Unlike BERT, GPT is unidirectional, reading text from left to right. However, its extensive pre-training on vast amounts of text data allows it to generate coherent and contextually relevant text that often mimics human writing.
Applications and Innovations
The applications of Transformers in NLP are vast and continue to expand. From improving search engine results to enabling more natural interactions with virtual assistants, Transformers are driving innovation across multiple domains.
Search Engines and Information Retrieval: One of the most visible impacts of Transformers is in search engines. Google, for instance, uses BERT to enhance its search algorithms, providing more accurate and relevant search results by understanding the context of queries better. This has transformed how we interact with search engines, making them more intuitive and responsive.
Virtual Assistants and Chatbots: Virtual assistants like Siri, Alexa, and Google Assistant have become integral parts of our daily lives. Transformers have significantly improved their ability to understand and generate natural language, making interactions more fluid and human-like. Chatbots powered by Transformers can handle complex customer service inquiries, provide personalized recommendations, and even engage in meaningful conversations.
Content Creation and Summarization: Transformers are revolutionizing content creation. Tools like GPT-4 can generate articles, reports, and even creative writing pieces that are coherent and engaging. They can also summarize long documents, extracting key information and presenting it concisely. This has immense potential in fields like journalism, where time and accuracy are crucial.
Language Translation: The ability of Transformers to capture context and meaning has also enhanced machine translation. Models like Google’s Multilingual Neural Machine Translation (MNMT) leverage Transformers to provide more accurate translations across multiple languages. This is breaking down language barriers and fostering global communication.
Sentiment Analysis and Opinion Mining: Businesses rely on sentiment analysis to gauge customer opinions and feedback. Transformers enable more accurate sentiment analysis by understanding the nuances of language. This helps companies tailor their products and services to better meet customer needs.
The Future of NLP with Transformers
As we look ahead, the future of NLP with Transformers appears incredibly promising. Several trends and developments are poised to shape this landscape further.
Multimodal Models: Combining text with other data forms, such as images and audio, is an exciting frontier. Multimodal models that integrate Transformers are being developed to handle diverse inputs, enhancing applications in areas like image captioning, video analysis, and audio-visual understanding.
Few-Shot and Zero-Shot Learning: Traditional machine learning models require vast amounts of labeled data for training. However, Transformers are pushing the boundaries with few-shot and zero-shot learning, where models can perform tasks with minimal or no task-specific training data. This opens up new possibilities for deploying NLP systems in resource-constrained environments.
Personalization and Adaptability: Future Transformer models will likely become more personalized, adapting to individual users’ preferences and needs. This could revolutionize fields like education, where personalized learning experiences can be created, and healthcare, where patient-specific recommendations can be generated.
Ethical Considerations: As Transformers become more powerful, ethical considerations become paramount. Issues such as bias, privacy, and the potential for misuse need to be addressed. Researchers and developers are working on frameworks and guidelines to ensure that these technologies are used responsibly and ethically.
Integration with Other AI Technologies: Transformers are increasingly being integrated with other AI technologies, such as reinforcement learning and computer vision. This convergence is leading to more sophisticated AI systems capable of performing a wide range of tasks with greater accuracy and efficiency.
Challenges and Limitations
Despite their impressive capabilities, Transformers are not without challenges and limitations.
Resource Intensive: Training and deploying Transformer models require significant computational resources and energy. This can be a barrier for smaller organizations and individuals looking to leverage this technology.
Bias and Fairness: Transformers can inadvertently learn and propagate biases present in training data. Ensuring fairness and mitigating bias is a critical challenge that researchers are actively addressing.
Interpretability: Understanding how Transformers make decisions is often complex due to their deep and intricate architectures. Developing methods to interpret and explain model outputs is an ongoing area of research.
Conclusion
Transformers have undeniably revolutionized the field of NLP, pushing the boundaries of what machines can understand and generate in human language. From enhancing search engines to powering virtual assistants, their impact is profound and far-reaching. As research and development continue, we can expect even more groundbreaking innovations that will further integrate Transformers into our daily lives.
The future of NLP with Transformers is not just about making machines understand language but making them do so with a depth of comprehension and creativity that was previously unimaginable. While challenges remain, the potential benefits of this technology are immense, promising a future where human and machine communication is more seamless and natural than ever before.
As we navigate this exciting landscape, it is essential to consider the ethical implications and work towards developing fair, transparent, and accountable AI systems. With responsible innovation, Transformers will undoubtedly continue to shape the future of NLP, unlocking new possibilities and transforming how we interact with the digital world.
Disclaimer: The information provided in this blog is based on current research and developments in the field of NLP and AI. It is subject to change as new advancements and discoveries are made. Please report any inaccuracies so we can correct them promptly.