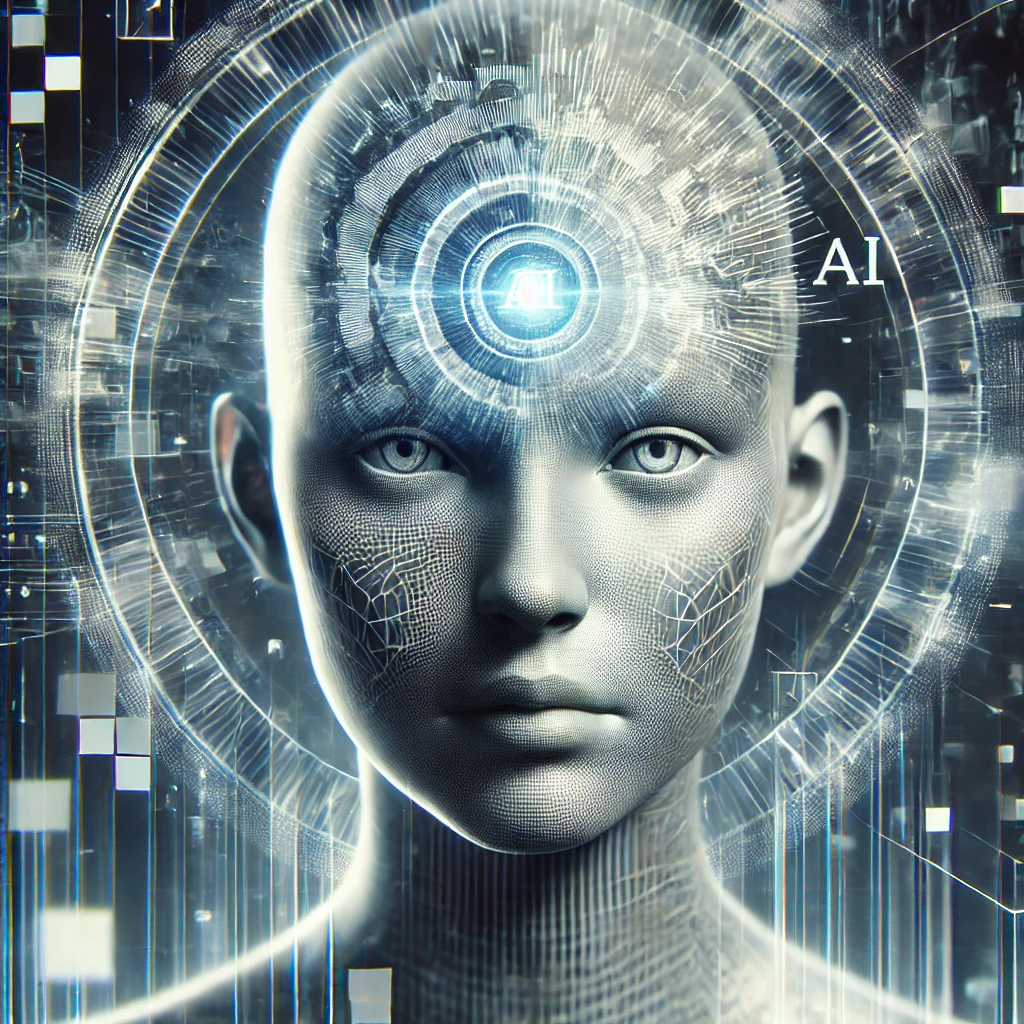
What Are AI Hallucinations and Can We Prevent Them?
In the rapidly evolving world of artificial intelligence, we’re constantly amazed by the capabilities of AI systems. They can write poetry, generate stunning artwork, and even engage in human-like conversations. But amidst all this progress, there’s a peculiar phenomenon that’s been catching the attention of researchers and users alike: AI hallucinations. It’s a term that sounds like it belongs in a sci-fi novel, but it’s very much a real issue in the AI landscape today. So, what exactly are these AI hallucinations, and more importantly, can we do anything to prevent them? Let’s dive into this fascinating and sometimes perplexing aspect of artificial intelligence.
Understanding AI Hallucinations
What are AI hallucinations?
AI hallucinations, despite their name, have nothing to do with the kind of hallucinations humans experience. Instead, this term refers to instances when an AI system generates or presents information that is entirely fabricated, nonsensical, or unrelated to the input it received. It’s as if the AI is “seeing” or “imagining” things that aren’t there in its training data or the given context. These hallucinations can range from small, inconsequential errors to major fabrications that can lead to significant misinformation if not caught and corrected.
Imagine asking an AI to write a biography of a famous historical figure, and suddenly it starts talking about that person’s adventures on Mars. Or perhaps you’re using an AI-powered image generator, and it adds an extra finger to every hand it draws. These are examples of AI hallucinations in action. They can be amusing at times, but they also highlight a serious challenge in the development of reliable AI systems.
Why do AI hallucinations occur?
To understand why AI hallucinations happen, we need to take a quick look at how these systems work. Most modern AI models, especially those used for language tasks, are based on what we call neural networks. These are complex mathematical models inspired by the human brain. They’re trained on vast amounts of data, learning patterns and relationships between different pieces of information.
When an AI model is asked to generate content or provide an answer, it doesn’t simply recall a pre-written response. Instead, it uses its understanding of patterns and relationships to construct a response that it thinks is most appropriate. This process is where hallucinations can creep in. If the model encounters a situation it’s not familiar with, or if there are gaps in its training data, it might fill in these gaps with information that seems plausible based on its learned patterns, but is actually incorrect or even completely made up.
Another factor contributing to AI hallucinations is the way these models are designed to be creative and generate novel content. This ability to be creative is what allows AI to write unique stories or come up with innovative solutions. However, this same feature can sometimes lead the AI to generate information that goes beyond what’s factually correct or contextually appropriate.
The Impact of AI Hallucinations
Why should we care about AI hallucinations?
AI hallucinations might seem like a minor issue at first glance. After all, humans make mistakes too, right? But when we consider the increasing reliance on AI systems in various fields, the potential impact of these hallucinations becomes clear. Let’s consider a few scenarios where AI hallucinations could have serious consequences.
In the field of healthcare, AI is being used to assist in diagnosing diseases and recommending treatments. If an AI system hallucinates and provides incorrect information about a patient’s condition or suitable medications, it could lead to serious health risks. Similarly, in financial sectors, AI systems are often used for risk assessment and investment recommendations. Hallucinations in this context could result in significant financial losses.
Even in less critical applications, like content creation or customer service chatbots, AI hallucinations can lead to the spread of misinformation or confusion among users. This can damage the reputation of companies using these AI tools and erode public trust in AI technology as a whole.
Real-world examples of AI hallucinations
To better understand the nature and impact of AI hallucinations, let’s look at some real-world examples. One notable instance occurred with ChatGPT, a popular language model developed by OpenAI. Users reported cases where ChatGPT confidently provided detailed information about nonexistent books, including fake titles, authors, and plot summaries. While this might seem harmless, imagine a student using this information for a book report or a researcher citing these non-existent sources in their work.
Another example comes from the legal field. In 2023, lawyers used ChatGPT to prepare a court filing, which included citations of several legal cases. It was later discovered that some of these cases were entirely fabricated by the AI. This incident led to significant embarrassment for the lawyers involved and raised serious questions about the use of AI in legal practice.
In the realm of image generation, AI hallucinations can manifest as visual anomalies. Users of image generation tools like DALL-E or Midjourney have often noticed strange artifacts in generated images, such as people with extra limbs or objects with impossible geometries. While these can sometimes be amusing, they highlight the potential for AI to produce unreliable visual content.
The Science Behind AI Hallucinations
How do neural networks lead to hallucinations?
To delve deeper into why AI hallucinations occur, we need to understand a bit more about how neural networks function. These networks are composed of layers of interconnected nodes, loosely inspired by the neurons in a human brain. During training, these networks adjust the strengths of connections between nodes to recognize patterns in the input data.
When a trained network is given a new input, it processes this information through its layers, with each layer extracting different features or aspects of the input. The final output is based on the network’s interpretation of these features. However, this process isn’t perfect. If the input contains elements that the network hasn’t encountered during training, or if it’s asked to generate information beyond its training data, it may produce outputs that don’t align with reality.
Moreover, the way these networks are trained can contribute to hallucinations. Many modern AI models use a technique called “self-supervised learning,” where they’re trained to predict missing parts of their input data. This allows them to learn from vast amounts of unlabeled data, but it can also lead them to “fill in the blanks” with plausible but incorrect information when faced with uncertainty.
The role of uncertainty in AI outputs
Uncertainty plays a crucial role in AI hallucinations. When humans are uncertain about something, we often express our doubt or qualify our statements. AI systems, however, don’t inherently have this ability. They’re designed to provide the most likely output based on their training, even when their “confidence” in that output is low.
This lack of uncertainty quantification means that an AI can provide a highly specific and detailed response even when it’s operating in areas where its knowledge is limited or nonexistent. To the user, this can appear as if the AI is stating facts, when in reality it’s generating plausible-sounding but potentially incorrect information.
Detecting AI Hallucinations
How can we identify AI hallucinations?
Identifying AI hallucinations can be challenging, especially for users who aren’t experts in the field or the subject matter the AI is addressing. However, there are several strategies we can employ to detect potential hallucinations:
- Fact-checking: Always verify important information provided by an AI against reliable sources. This is particularly crucial for factual claims, dates, statistics, or any information that could have real-world consequences if incorrect.
- Look for inconsistencies: AI hallucinations often manifest as information that contradicts itself or doesn’t align with well-established facts. If you notice such inconsistencies, it’s a red flag that the AI might be hallucinating.
- Be wary of overly specific details: If an AI provides extremely specific details about a topic, especially if it’s a complex or obscure one, it might be a sign of hallucination. AI models often generate such details to make their outputs seem more plausible.
- Use multiple AI models: If possible, cross-reference information across different AI models. If they provide conflicting information, it’s a sign that at least one of them might be hallucinating.
- Consider the context: If the AI provides information that seems out of place given the context of your query or the known capabilities of the model, it could be a hallucination.
Tools and techniques for hallucination detection
As AI hallucinations become a more recognized issue, researchers and developers are working on tools to help detect them. Some of these approaches include:
- Confidence scoring: Some AI systems are being developed to provide a confidence score along with their outputs. This score indicates how certain the AI is about the information it’s providing.
- Explainable AI: This involves developing AI systems that can provide explanations for their outputs. By understanding the reasoning behind an AI’s response, it may be easier to spot potential hallucinations.
- Fact-checking AI: These are AI systems specifically designed to verify claims made by other AI models by cross-referencing them against large databases of verified information.
- Adversarial testing: This involves intentionally giving AI systems inputs designed to provoke hallucinations, helping to identify weak points in the model’s knowledge or reasoning.
Preventing AI Hallucinations
Can we completely prevent AI hallucinations?
The million-dollar question is: can we completely prevent AI hallucinations? The short answer, unfortunately, is probably not. At least, not with our current AI technologies. The very nature of how these AI models work โ generating responses based on patterns in their training data rather than a true understanding of the world โ means that there will always be a potential for hallucinations.
However, this doesn’t mean we’re powerless against AI hallucinations. There are several strategies being explored and implemented to minimize their occurrence and impact.
Strategies for minimizing hallucinations
- Improved training data: One of the most straightforward approaches is to improve the quality and breadth of the data used to train AI models. By exposing the AI to more diverse and accurate information during training, we can reduce the likelihood of it generating false information.
- Fine-tuning and specialized models: Rather than relying on general-purpose AI models for all tasks, developing and fine-tuning models for specific domains can help reduce hallucinations in those areas. For example, a model specifically trained on medical literature would be less likely to hallucinate when answering health-related questions.
- Implementing fact-checking mechanisms: Building in automated fact-checking processes that cross-reference AI outputs against verified databases can help catch and filter out hallucinations before they reach the user.
- Uncertainty quantification: Developing AI models that can express uncertainty about their outputs could help users identify potential hallucinations. This might involve the AI providing confidence scores or clearly stating when it’s extrapolating beyond its training data.
- Human-AI collaboration: In many cases, the most effective approach is to use AI as a tool to augment human intelligence rather than replace it entirely. Having human experts review and verify AI outputs can significantly reduce the impact of hallucinations.
The Future of AI and Hallucinations
Ongoing research and development
The challenge of AI hallucinations is at the forefront of AI research. Scientists and developers are continually working on new approaches to make AI systems more reliable and less prone to hallucinations. Some areas of ongoing research include:
- Causal AI: This involves developing AI systems that understand cause-and-effect relationships rather than just statistical correlations. This could lead to more robust reasoning and fewer hallucinations.
- Neuro-symbolic AI: This approach combines neural networks with symbolic AI, which uses logical rules and knowledge representation. The goal is to create AI systems that can reason more like humans, potentially reducing hallucinations.
- Large language models with external knowledge bases: By connecting AI models to regularly updated external knowledge bases, we might be able to reduce hallucinations and keep the AI’s knowledge current.
- Adversarial training: This involves intentionally exposing AI models to inputs designed to cause hallucinations during training, helping them become more robust against such issues.
The ethical implications of AI hallucinations
As we continue to develop and deploy AI systems, it’s crucial to consider the ethical implications of AI hallucinations. These issues raise important questions about transparency, accountability, and trust in AI technologies.
For instance, who is responsible when an AI hallucination leads to harm? The developers of the AI? The company deploying it? The user who didn’t fact-check the AI’s output? These are complex questions that our society will need to grapple with as AI becomes more integrated into our daily lives.
Moreover, the potential for AI hallucinations highlights the importance of maintaining human oversight and critical thinking skills. As we become more reliant on AI systems, we must ensure that we don’t lose our ability to question and verify information, regardless of its source.
Conclusion
AI hallucinations are a fascinating and challenging aspect of artificial intelligence. They remind us that while AI has made incredible strides, it’s still far from perfect. These systems, no matter how advanced, are ultimately based on pattern recognition and statistical correlations, not true understanding.
As we continue to develop and deploy AI technologies, it’s crucial that we remain aware of the potential for hallucinations. We must approach AI outputs with a critical eye, always ready to verify important information. At the same time, we should be excited about the ongoing research and development in this field. The challenges posed by AI hallucinations are driving innovations that could lead to more reliable, trustworthy, and capable AI systems in the future.
Ultimately, the goal isn’t to create AI that never makes mistakes โ even humans can’t achieve that. Instead, we’re working towards AI systems that can work alongside humans, augmenting our capabilities while being transparent about their limitations and uncertainties. As we navigate this journey, it’s an exciting time to be involved in or simply observing the world of artificial intelligence.
Disclaimer: This article aims to provide an overview of AI hallucinations based on current understanding and research. As this is a rapidly evolving field, some information may become outdated over time. We encourage readers to consult recent scientific literature and expert opinions for the most up-to-date information on this topic. If you notice any inaccuracies in this article, please report them so we can correct them promptly.